Semi-supervised image clustering subspace learning algorithm based on local linear regression
A subspace learning and image clustering technology, applied in computing, computer parts, instruments, etc., can solve problems such as comparable accuracy
- Summary
- Abstract
- Description
- Claims
- Application Information
AI Technical Summary
Problems solved by technology
Method used
Image
Examples
Embodiment 1
[0087] A semi-supervised image clustering subspace learning algorithm based on local linear regression, such as figure 1 As shown, the specific steps are as follows:
[0088] The first step, the prediction error of the feature vector x
[0089] (1) Construct a training data set, including labeled samples and unlabeled samples;
[0090] (2) For each image in the training data set, extract the underlying color, texture and shape features, specifically including HSV color histogram, color aggregation vector and Tamura orientation, to form a feature vector x.
[0091] (3) Use the local linear regression method to predict the coordinates of the feature vector x in the clustering subspace, and obtain the predicted value
[0092] z=ψ T x+ξ(1)
[0093] In formula (1): ψ represents the regression matrix;
[0094] ξ means bias;
[0095] T represents the transpose operation.
[0096] (4) Suppose the optimal value of the coordinates of the feature vector x in the clustering subspac...
Embodiment 2
[0159] A semi-supervised image clustering subspace learning algorithm based on local linear regression, the specific steps are as follows:
[0160] The first step, the prediction error of the feature vector x
[0161] (1) Collect images of six categories including tiger, car, explosion, bird, lightning and dolphin to form a training data set, including labeled samples and unlabeled samples, where each category contains 300 images.
[0162] (2) For each image in the training data set, extract the underlying color, texture and shape features, specifically including HSV color histogram, color aggregation vector and Tamura orientation, to form a feature vector x.
[0163] (3) Use the local linear regression method to predict the coordinates of the feature vector x in the clustering subspace, and obtain the predicted value
[0164] z=ψ T x+ξ(1)
[0165] In formula (1): ψ represents the regression matrix;
[0166] ξ means bias;
[0167] T represents the transpose operation.
...
PUM
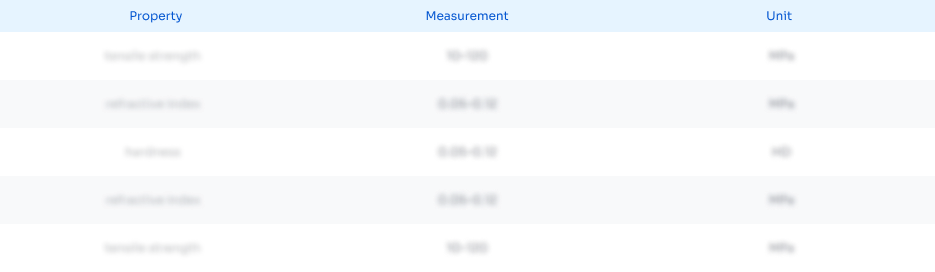
Abstract
Description
Claims
Application Information
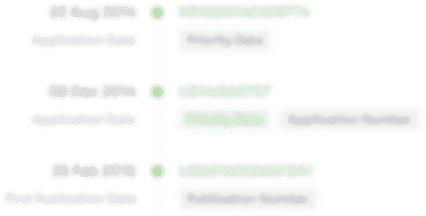
- R&D
- Intellectual Property
- Life Sciences
- Materials
- Tech Scout
- Unparalleled Data Quality
- Higher Quality Content
- 60% Fewer Hallucinations
Browse by: Latest US Patents, China's latest patents, Technical Efficacy Thesaurus, Application Domain, Technology Topic, Popular Technical Reports.
© 2025 PatSnap. All rights reserved.Legal|Privacy policy|Modern Slavery Act Transparency Statement|Sitemap|About US| Contact US: help@patsnap.com