Positive semidefinite spectral clustering method based on Lagrange dual
A semi-positive definite, spectral clustering technique, applied in the field of spectral clustering
- Summary
- Abstract
- Description
- Claims
- Application Information
AI Technical Summary
Problems solved by technology
Method used
Image
Examples
Embodiment Construction
[0051] Below in conjunction with accompanying drawing and embodiment the method of the present invention is described in detail, present embodiment is carried out under the premise of technical scheme of the present invention, has provided embodiment and specific operation process, but protection scope of the present invention is not limited to following the embodiment.
[0052] The implementation of the embodiment of the present invention includes the following steps:
[0053] S1. Given a sample data set {(a 1 ,...,a n )|a i ∈R M ,i=1,...,n}. a i Represents the feature vector of the i-th sample data; the dimension of each feature vector is M (M is a natural number); n is the number of samples (n is a natural number) and the order of magnitude is 10 3 above. The number of categories contained in the sample data set is k (k is a natural number). The number of categories generally ranges from 1 to 100. The dimensionality of the data was reduced using principal component ...
PUM
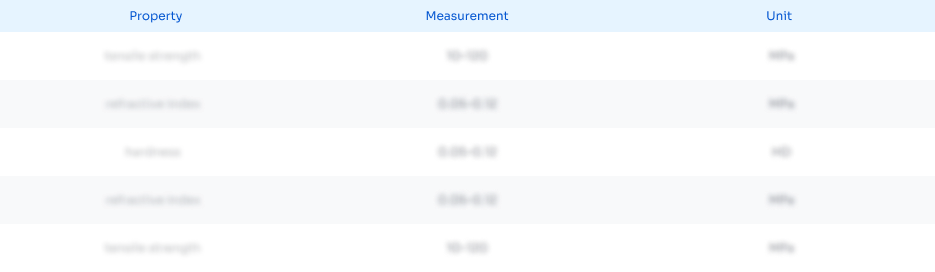
Abstract
Description
Claims
Application Information
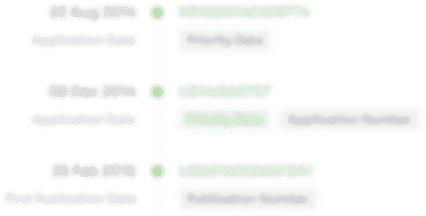
- R&D Engineer
- R&D Manager
- IP Professional
- Industry Leading Data Capabilities
- Powerful AI technology
- Patent DNA Extraction
Browse by: Latest US Patents, China's latest patents, Technical Efficacy Thesaurus, Application Domain, Technology Topic, Popular Technical Reports.
© 2024 PatSnap. All rights reserved.Legal|Privacy policy|Modern Slavery Act Transparency Statement|Sitemap|About US| Contact US: help@patsnap.com