Remote sensing image change detection method based on controllable kernel regression and superpixel segmentation
A superpixel segmentation and remote sensing image technology, applied in the field of image processing, can solve the problems of noise sensitivity, missed detection, and low accuracy of final detection results, and achieve the effect of improving the accuracy rate, reducing the total number of errors, and suppressing noise.
- Summary
- Abstract
- Description
- Claims
- Application Information
AI Technical Summary
Problems solved by technology
Method used
Image
Examples
Embodiment Construction
[0024] refer to figure 1 ,本发明的具体实现步骤如下:
[0025] 步骤1,输入同一地区不同时间获取的已配准的两幅遥感图像X 1 and x 2 .
[0026] 输入同一地区获取的已配准的两幅大小均为I×J的两时相遥感图像:第一时相图像为X 1 ={X 1 (i,j)|1≤i≤I,1≤j≤J},第二时相图像为X 2 ={X 2(i,j)|1≤i≤I,1≤j≤J}, where X 1 (i,j) and X 2 (i, j) are the pixel gray value of the first phase image and the second phase image at the spatial position (i, j) respectively, i and j are the row number and column number of the image respectively, and I is the image The total number of rows, J is the total number of columns of the image.
[0027] Step 2, calculate the first phase image X 1 and the second phase image X 2 The structural feature matrix W S1 and W S2 .
[0028] The methods for calculating the structural feature matrix include nonlinear adaptive interpolation method, anisotropic directional diffusion PDE method, controllable kernel regression method, etc. The controllable kernel regression method given in this embodiment has the following specific steps:
[0029] 2a) The fir...
PUM
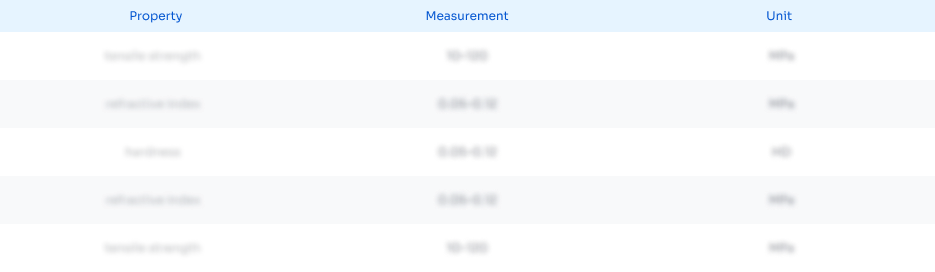
Abstract
Description
Claims
Application Information
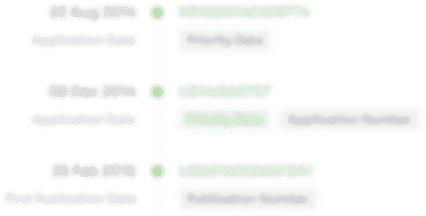
- Generate Ideas
- Intellectual Property
- Life Sciences
- Materials
- Tech Scout
- Unparalleled Data Quality
- Higher Quality Content
- 60% Fewer Hallucinations
Browse by: Latest US Patents, China's latest patents, Technical Efficacy Thesaurus, Application Domain, Technology Topic, Popular Technical Reports.
© 2025 PatSnap. All rights reserved.Legal|Privacy policy|Modern Slavery Act Transparency Statement|Sitemap|About US| Contact US: help@patsnap.com