Change Detection Method of Remote Sensing Image Based on Steerable Kernel Regression and Superpixel Segmentation
A super-pixel segmentation and remote sensing image technology, which is applied in the field of image processing, can solve the problems of low accuracy rate, missed detection, and noise sensitivity of the final detection results, and achieve the effects of suppressing noise, reducing the total number of errors, and improving the accuracy rate
- Summary
- Abstract
- Description
- Claims
- Application Information
AI Technical Summary
Problems solved by technology
Method used
Image
Examples
Embodiment Construction
[0024] refer to figure 1 , the specific implementation steps of the present invention are as follows:
[0025] Step 1, input two registered remote sensing images X obtained at different times in the same area 1 and x 2 .
[0026] Input two registered two-temporal remote sensing images of size I×J acquired in the same area: the first temporal image is X 1 ={X 1 (i,j)|1≤i≤I, 1≤j≤J}, the second phase image is X 2 ={X 2(i,j)|1≤i≤I,1≤j≤J}, where X 1 (i,j) and X 2 (i, j) are the pixel gray value of the first phase image and the second phase image at the spatial position (i, j) respectively, i and j are the row number and column number of the image respectively, and I is the image The total number of rows, J is the total number of columns of the image.
[0027] Step 2, calculate the first phase image X 1 and the second phase image X 2 The structural feature matrix W S1 and W S2 .
[0028] The methods for calculating the structural feature matrix include nonlinear adapti...
PUM
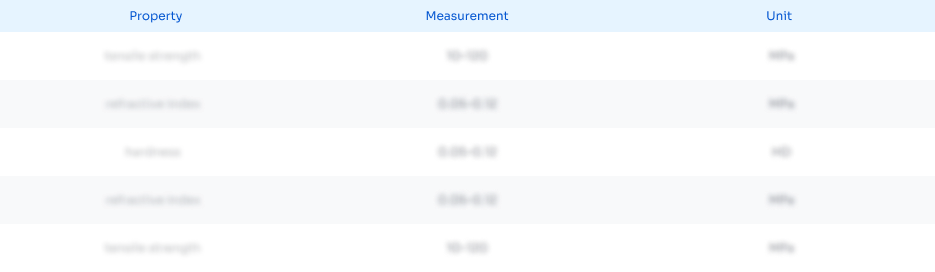
Abstract
Description
Claims
Application Information
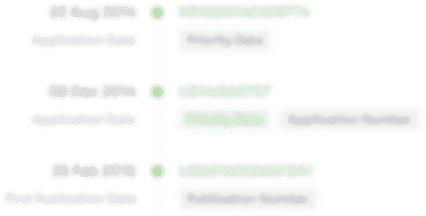
- R&D Engineer
- R&D Manager
- IP Professional
- Industry Leading Data Capabilities
- Powerful AI technology
- Patent DNA Extraction
Browse by: Latest US Patents, China's latest patents, Technical Efficacy Thesaurus, Application Domain, Technology Topic.
© 2024 PatSnap. All rights reserved.Legal|Privacy policy|Modern Slavery Act Transparency Statement|Sitemap