Method for finely classifying polarized SAR images based on Freeman entropy and self-learning
A fine classification and classification method technology, applied in the field of image processing, can solve the problems of high cost of manually defining labels, difficulty in obtaining labels, slow convergence speed, etc., and achieve the effect of solving shadow confusion
- Summary
- Abstract
- Description
- Claims
- Application Information
AI Technical Summary
Problems solved by technology
Method used
Image
Examples
Embodiment Construction
[0033] Reference figure 1 The specific implementation steps of the present invention are as follows:
[0034] Step 1: Perform eigenvalue decomposition on all pixels of the polarized SAR image G.
[0035] The information of each pixel of the polarization SAR data is represented by a polarization coherence matrix T with a size of 3×3. Since the eigenvalues of the matrix can best represent the information contained in the matrix, the eigs function of MATLAB is used to perform eigen decomposition on the polarization coherence matrix T of each pixel. The decomposition expression is as follows:
[0036] [ T ] = [ U 3 ] λ 1 0 0 0 λ 2 0 0 0 λ 3 [ U 3 ] T ,
[0037] Where U 3 Is the eigenvector of the polarization coherence matrix T eigenvalue decomposition, λ 1 ,λ 2 ,λ 3 The eigenvalues of different sizes obtained by eigenvalue decomposition of the polarization coherence matrix T are ordere...
PUM
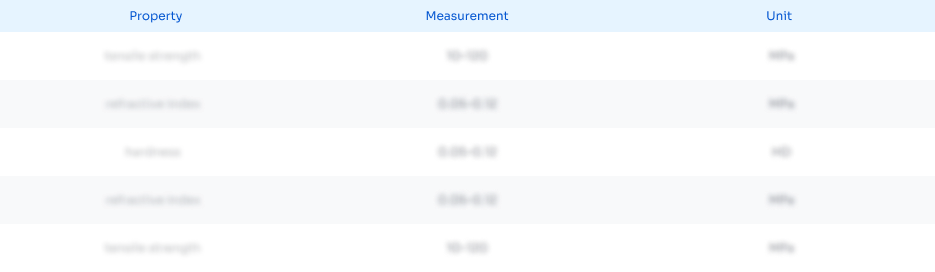
Abstract
Description
Claims
Application Information
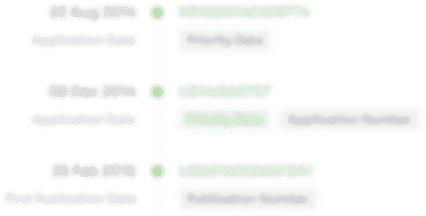
- R&D Engineer
- R&D Manager
- IP Professional
- Industry Leading Data Capabilities
- Powerful AI technology
- Patent DNA Extraction
Browse by: Latest US Patents, China's latest patents, Technical Efficacy Thesaurus, Application Domain, Technology Topic, Popular Technical Reports.
© 2024 PatSnap. All rights reserved.Legal|Privacy policy|Modern Slavery Act Transparency Statement|Sitemap|About US| Contact US: help@patsnap.com