Fast video key frame extraction method of principal component characteristic curve analysis
A technology of video key frames and characteristic curves, applied in image analysis, image data processing, instruments, etc., can solve the problems of inability to accurately extract key frames and good algorithm effects
- Summary
- Abstract
- Description
- Claims
- Application Information
AI Technical Summary
Problems solved by technology
Method used
Image
Examples
Embodiment 1
[0033] For a natural landscape video sequence containing 11096 frames, follow the method described above:
[0034]1) Extract four image visual features of SIFT, HOG, GIST and PHOI from the video frame image, a total of 500+576+512+2040=3628-dimensional feature expression, splicing the above four types of image visual features in sequence to get The characteristic matrix X=[x that this video sequence image frame characteristic constitutes 1 ,x 2 ,...,x n ]∈R d×n , where d is 3628 dimensions, n=11096;
[0035] 2) Perform PCA dimension reduction processing on the feature matrix X to obtain a 500-dimensional image low-dimensional feature expression Y=W T X=[y 1 ,y 2 ,...,y n ]∈R m×n , where y i is the low-dimensional feature expression corresponding to the i-th frame of the video sequence;
[0036] 3) The low-dimensional feature expression Y of the image is regarded as m principal component characteristic curves that change with time series, and each principal component ...
Embodiment 2
[0039] For a Tai Chi motion video sequence that includes 12444 frames, follow the method described above:
[0040] 4) Extract four image visual features of SIFT, HOG, GIST and PHOI from the video frame image, totaling 500+576+512+2040=3628 dimensional feature expression, splicing the above four types of image visual features in sequence to get The characteristic matrix X=[x that this video sequence image frame characteristic constitutes 1 ,x 2 ,...,x n ]∈R d×n , where d is 3628 dimensions, n=12444;
[0041] 5) Perform PCA dimension reduction processing on the feature matrix X to obtain a 500-dimensional image low-dimensional feature expression Y=W T X=[y 1 ,y 2 ,...,y n ]∈R m×n , where y i is the low-dimensional feature expression corresponding to the i-th frame of the video sequence;
[0042] 6) The low-dimensional feature expression Y of the image is regarded as m principal component characteristic curves that change with time series, and each principal component c...
Embodiment 3
[0045] For a BBC news video sequence containing 2340 frames, follow the method described above:
[0046] 7) Extract four image visual features of SIFT, HOG, GIST and PHOI from the video frame image, a total of 500+576+512+2040=3628-dimensional feature expression, splicing the above four types of image visual features in sequence to get The characteristic matrix X=[x that this video sequence image frame characteristic constitutes 1 ,x 2 ,...,x n ]∈R d×n , where d is 3628 dimensions, n=2340;
[0047] 8) Perform PCA dimension reduction processing on the feature matrix X to obtain a 500-dimensional image low-dimensional feature expression Y=W T X=[y 1 ,y 2 ,...,y n ]∈R m×n , where y i is the low-dimensional feature expression corresponding to the i-th frame of the video sequence;
[0048] 9) The low-dimensional feature expression Y of the image is regarded as m principal component characteristic curves that change with time series, and each principal component characteri...
PUM
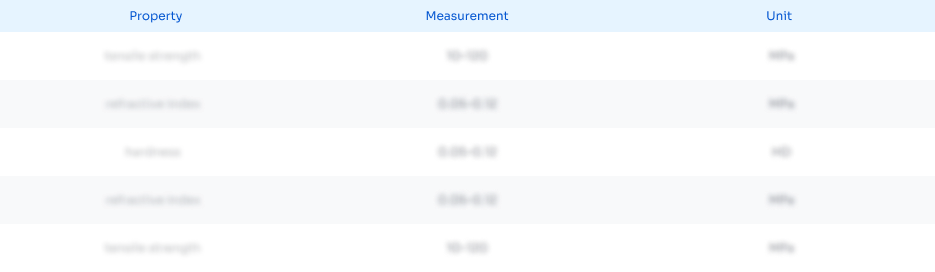
Abstract
Description
Claims
Application Information
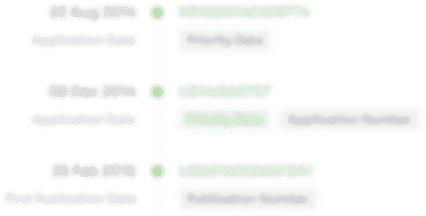
- R&D Engineer
- R&D Manager
- IP Professional
- Industry Leading Data Capabilities
- Powerful AI technology
- Patent DNA Extraction
Browse by: Latest US Patents, China's latest patents, Technical Efficacy Thesaurus, Application Domain, Technology Topic, Popular Technical Reports.
© 2024 PatSnap. All rights reserved.Legal|Privacy policy|Modern Slavery Act Transparency Statement|Sitemap|About US| Contact US: help@patsnap.com