Data-driven and task-driven image classification method
A task-driven, classification method technology, applied in the direction of instruments, biological neural network models, character and pattern recognition, etc., can solve problems that are not utilized, not optimal, etc., and achieve efficient training, good performance, and efficient K-nearest neighbor image classification Effect
- Summary
- Abstract
- Description
- Claims
- Application Information
AI Technical Summary
Problems solved by technology
Method used
Image
Examples
Embodiment Construction
[0044] In order to make the object, technical solution and advantages of the present invention clearer, the present invention will be described in further detail below in conjunction with specific embodiments and with reference to the accompanying drawings.
[0045] The idea of the present invention is: 1) Based on nonlinear convolution feature learning, the model can be adaptive to the data set in a data-driven manner, so as to better describe a specific data set; 2) the present invention directly uses K The error of the nearest neighbor is optimized, and the convolutional neural network is optimized in a task-driven manner, so that it can achieve better performance on the K-nearest neighbor task; 3) In the training phase, GPU can be used for efficient training, and in the testing phase, only Efficient K-nearest neighbor image classification can be achieved by using CPU, which is very suitable for large-scale image classification, retrieval and other tasks.
[0046] like f...
PUM
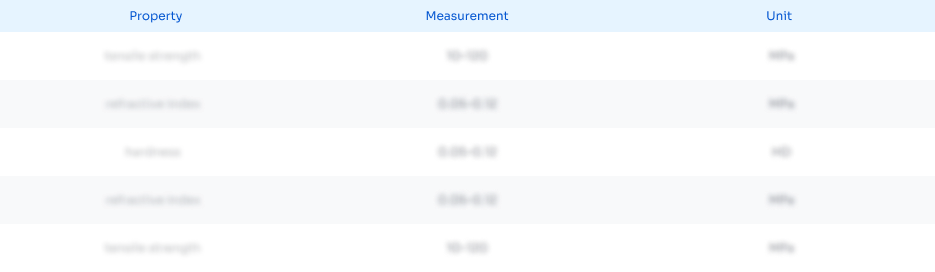
Abstract
Description
Claims
Application Information
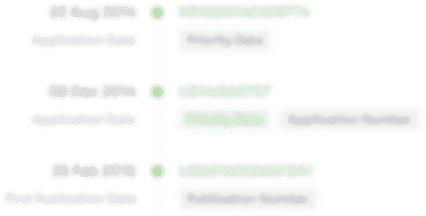
- R&D Engineer
- R&D Manager
- IP Professional
- Industry Leading Data Capabilities
- Powerful AI technology
- Patent DNA Extraction
Browse by: Latest US Patents, China's latest patents, Technical Efficacy Thesaurus, Application Domain, Technology Topic, Popular Technical Reports.
© 2024 PatSnap. All rights reserved.Legal|Privacy policy|Modern Slavery Act Transparency Statement|Sitemap|About US| Contact US: help@patsnap.com