BP neural network-based ultra-short load prediction method
A load forecasting, neural network technology, applied in neural learning methods, biological neural network models, forecasting, etc., can solve problems such as the reduction of load forecasting accuracy
- Summary
- Abstract
- Description
- Claims
- Application Information
AI Technical Summary
Problems solved by technology
Method used
Image
Examples
Embodiment Construction
[0054] The present invention will be described in detail below in conjunction with the accompanying drawings.
[0055] This method is based on taking the load data of 96 points on a certain day in a certain city as a sample, the daily information d is an integer from 1 to 7, and the cycle is weekly. Specific steps such as figure 1 Shown:
[0056] Step 1 Perform microgrid data preprocessing
[0057] (1) Mutation data identification, according to the following formula
[0058]
[0059] L d,t is the load value at time t of day d; u=0.01 is the judgment threshold; M is the last moment of day d-1. When the load change rate at adjacent moments is greater than the judgment threshold, the load data L d,t It is the mutation data and is eliminated, and then the data at this moment is completed as the missing data;
[0060] (2) Incomplete data use the following formula
[0061]
[0062] L d-m,t is the load data at time t of day d-m; λ is the weight coefficient, which means L ...
PUM
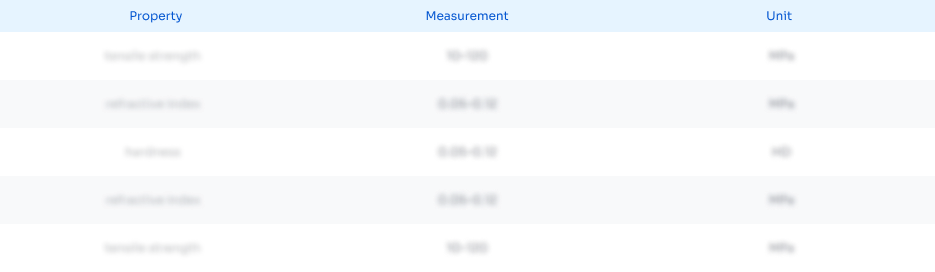
Abstract
Description
Claims
Application Information
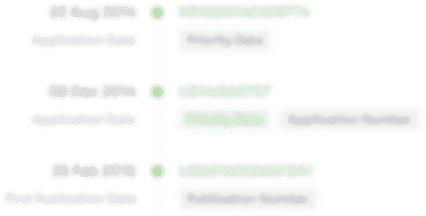
- R&D Engineer
- R&D Manager
- IP Professional
- Industry Leading Data Capabilities
- Powerful AI technology
- Patent DNA Extraction
Browse by: Latest US Patents, China's latest patents, Technical Efficacy Thesaurus, Application Domain, Technology Topic, Popular Technical Reports.
© 2024 PatSnap. All rights reserved.Legal|Privacy policy|Modern Slavery Act Transparency Statement|Sitemap|About US| Contact US: help@patsnap.com