Categorizing method oriented to Internet unbalanced application flow
A classification method and application flow technology, which is applied in special data processing applications, instruments, electrical digital data processing, etc., can solve the problems of low classification accuracy of small categories, unbalanced application flow, and low overall byte classification accuracy
- Summary
- Abstract
- Description
- Claims
- Application Information
AI Technical Summary
Problems solved by technology
Method used
Image
Examples
Embodiment 1
[0038] A classification method for unbalanced Internet application flow of the present invention includes 5 steps, which are divided into three parts: flow data preprocessing, such as S101, S102 and S103; off-line training of the flow classification model, such as S104; flow classification S105.
[0039] S101, using the k-means algorithm to divide the data set into multiple dense and disjoint subsets, each subset contains a cluster center;
[0040] S102: For the subset obtained in S101, expand the small class flow samples according to the oversampling ratio;
[0041] S103: Aiming at the subset obtained in S102, formulate a heuristic rule to undersample the large class flow samples;
[0042] S104: Using the subset obtained in S103 as a training set, train k integrated classification models offline;
[0043] S105: Combine k integrated classification models to classify the test stream samples.
Embodiment 2
[0045] S101, using the k-means clustering algorithm to divide the traffic data set into multiple dense and disjoint data subsets, the number of clusters k is determined by: using the sum of squared error (SSE) search k value, SSE represents the sum of the divergence within each cluster when the data set is divided into k clusters, the calculation of the sum of the SSE of each cluster is as formula (1), where x i Denotes the i-th flow sample, μ j Indicates the jth cluster center, n j Indicates the number of flow samples of the jth cluster;
[0046] SSE = Σ j = 1 k Σ i = 1 n j ( x i - μ j ) ...
Embodiment 3
[0052] S102, the classification method for Internet unbalanced application flow is characterized in that expanding the small flow sample according to the oversampling ratio includes the flow of the oversampling ratio and expanding the small flow sample; the "oversampling ratio" refers to the In the oversampling subset, the ratio of the number of flow samples of the largest class to the number of flow samples of a small class is set artificially; the oversampling subset is a flow sample data set obtained by expanding the flow samples of the small class in the current subset ; The current subset contains samples of multiple categories, which are divided into three parts: samples of 1 largest class, samples of 1 or more small classes, and samples of 1 or more other classes; the largest class refers to The category with the largest number of samples in the current subset; the small category refers to the category that expands at least one sample in the current subset and satisfies ...
PUM
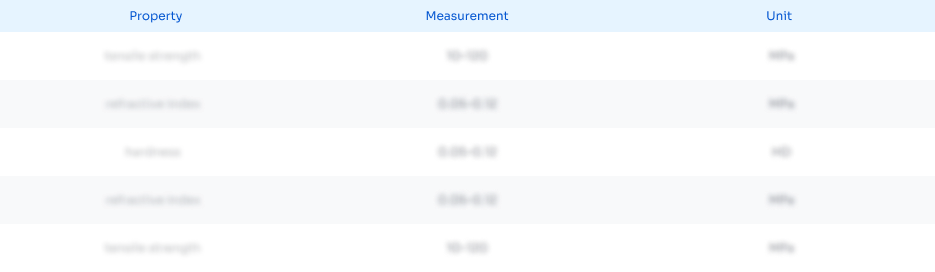
Abstract
Description
Claims
Application Information
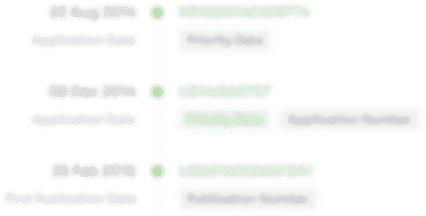
- R&D
- Intellectual Property
- Life Sciences
- Materials
- Tech Scout
- Unparalleled Data Quality
- Higher Quality Content
- 60% Fewer Hallucinations
Browse by: Latest US Patents, China's latest patents, Technical Efficacy Thesaurus, Application Domain, Technology Topic, Popular Technical Reports.
© 2025 PatSnap. All rights reserved.Legal|Privacy policy|Modern Slavery Act Transparency Statement|Sitemap|About US| Contact US: help@patsnap.com