Hyperspectral remote sensing data classification method based on deep learning
A hyperspectral remote sensing and deep learning technology, applied in the field of hyperspectral data classification, can solve the problem of low classification accuracy, achieve accurate data classification and reduce the effect of impact
- Summary
- Abstract
- Description
- Claims
- Application Information
AI Technical Summary
Problems solved by technology
Method used
Image
Examples
specific Embodiment approach 1
[0033] Specific implementation mode one: the following combination figure 1 Describe this embodiment, the hyperspectral remote sensing data classification method based on deep learning described in this embodiment, it comprises the following steps:
[0034] Step 1: Read the hyperspectral raw data, use the principal component analysis method to obtain the eigenvalues and eigenvectors of the hyperspectral raw data, and then obtain the spectral eigenvectors of the hyperspectral raw data; Domain information extraction to obtain spatial feature information of hyperspectral raw data;
[0035] Step 2: Integrate the spectral feature vector and spatial feature information of the hyperspectral raw data to obtain hyperspectral integrated data;
[0036] Step 3: Determine the labeled samples from the hyperspectral integrated data, and select training samples and test samples from the labeled samples;
[0037] Step 4: Based on the deep learning method, use the training samples to pre-tr...
specific Embodiment approach 2
[0040] Specific implementation mode 2: This implementation mode further explains the implementation mode 1, and the acquisition method of the spectral feature vector of the hyperspectral raw data is as follows:
[0041] First calculate the covariance matrix of the hyperspectral raw data, and use the principal component analysis method to calculate the eigenvalues and eigenvectors of all the hyperspectral raw data according to the covariance matrix, and arrange the corresponding features according to the order of the eigenvalues from large to small Vector; use eigenvectors as weighting coefficients to calculate and obtain B band principal component components of all eigenvectors, and use all eigenvectors containing B band principal component components as spectral eigenvectors of hyperspectral raw data; B is a positive integer;
[0042] The method to obtain the spatial feature information of hyperspectral raw data is:
[0043] Select the first N principal component componen...
specific Embodiment approach 3
[0047] Specific implementation mode three: this implementation mode further explains implementation mode two, and the specific method for obtaining hyperspectral integrated data is: the pixel point (x i ,y i ) is regarded as a spectral feature vector of length B, and all pixels at the same coordinate position in the hyperspectral raw data (x i ,y i ), a spectral eigenvector of length B and a length of N×w 2 The spatial feature information of is integrated into a length of (B+N×w 2 ) vector, as hyperspectral integration data.
[0048] In this embodiment, after information integration of hyperspectral data, each pixel will have a length (B+N×w) containing spectral information and spatial information 2 ) eigenvectors.
PUM
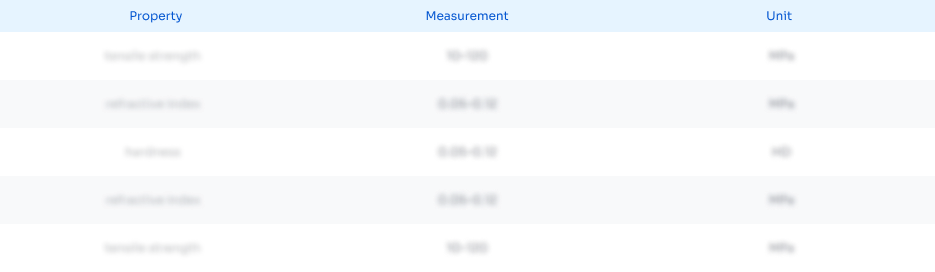
Abstract
Description
Claims
Application Information
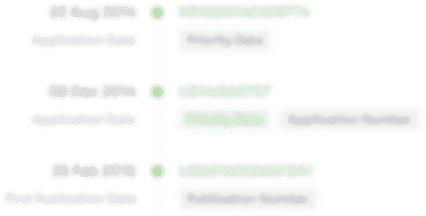
- Generate Ideas
- Intellectual Property
- Life Sciences
- Materials
- Tech Scout
- Unparalleled Data Quality
- Higher Quality Content
- 60% Fewer Hallucinations
Browse by: Latest US Patents, China's latest patents, Technical Efficacy Thesaurus, Application Domain, Technology Topic, Popular Technical Reports.
© 2025 PatSnap. All rights reserved.Legal|Privacy policy|Modern Slavery Act Transparency Statement|Sitemap|About US| Contact US: help@patsnap.com