LS-SVM-based method for detecting anomaly slot of sensor detection data
A technology for detecting data and detection methods, which is applied in electrical digital data processing, special data processing applications, instruments, etc., and can solve problems such as difficulty in judging short-term trends or pattern changes or anomalies in time series
- Summary
- Abstract
- Description
- Claims
- Application Information
AI Technical Summary
Problems solved by technology
Method used
Image
Examples
specific Embodiment approach 1
[0018] Specific implementation mode one: see figure 1 Describe this embodiment, a kind of sensor detection data abnormal segment detection method based on LS-SVM described in this embodiment, it comprises the following steps:
[0019] Step 1. Set the required detection confidence c, the time series length n and the minimum number of abnormal points m, and the settings of n and m meet the limits of the confidence c, and both n and m are positive integers;
[0020] Step two, from t 0 From time to time, point anomaly detection is performed on the data within the time series length n, and the LS-SVM point anomaly detection with a confidence probability of p is obtained to obtain the number of prediction residuals and data abnormal points within the time series length n;
[0021] Step 3. Determine whether the number of abnormal points in the time series of length n in step 2 is at least m abnormal points, that is, |E n (t 0 )|≥m, m is a positive integer, |E n (t 0 )|represents...
specific Embodiment approach 2
[0030] Specific embodiment 2: This embodiment is a further description of the method for detecting abnormal fragments of sensor detection data based on LS-SVM described in specific embodiment 1. In this embodiment, in step 1, the time series length n, minimum abnormal The number m of points and the degree of confidence c, and the relationship between the three satisfies the following formula:
[0031] P(|E n (t 0 )|≥m)>c,
[0032] Among them, in a time series segment of length n, the probability P(|E n (t 0 )|≥m) is expressed as P(|E n (t 0 )|≥m)=P(m)+P(m+1)+…+P(n), P(m) is the probability of m outliers appearing in the time series length of n, P(|E n (t 0 )|) is the occurrence of |E in a time series segment of length n n (t 0 )|the probability of an outlier, P ( | E n ( t 0 ) | ...
specific Embodiment approach 3
[0035] Specific embodiment three, this embodiment is a kind of LS-SVM-based sensor detection data abnormal segment detection method described in specific embodiment one to further explain, in this embodiment, step two from t 0 From time to time, point anomaly detection is performed on the data within the time series length n with the LS-SVM point anomaly detection with a confidence probability of p. The method to obtain the number of abnormal points of the data within the time series length n is:
[0036] Step 21: Set the training data set, perform phase space reconstruction on the data in the training data set, and obtain the input vector and output vector;
[0037] Step 22: Using the Z-zeros method to normalize the obtained input vector and output vector, and normalize the input vector and output vector to a range of [-1, 1];
[0038] Step two and three: select the kernel function of the LS-SVM algorithm, and set the parameters of the LS-SVM prediction model, and train the L...
PUM
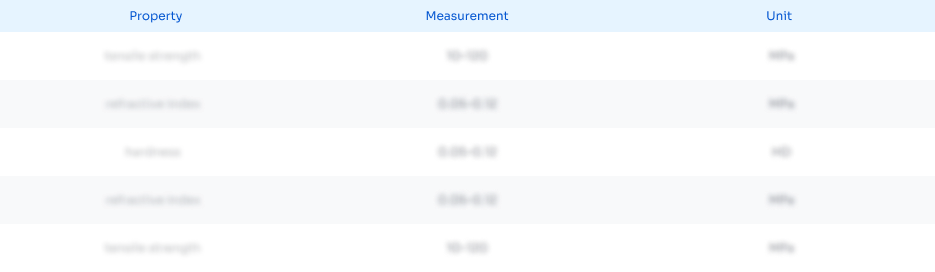
Abstract
Description
Claims
Application Information
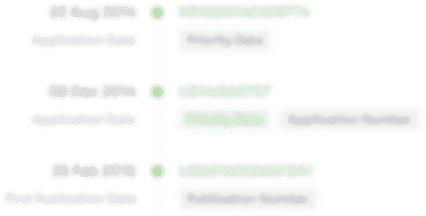
- R&D Engineer
- R&D Manager
- IP Professional
- Industry Leading Data Capabilities
- Powerful AI technology
- Patent DNA Extraction
Browse by: Latest US Patents, China's latest patents, Technical Efficacy Thesaurus, Application Domain, Technology Topic, Popular Technical Reports.
© 2024 PatSnap. All rights reserved.Legal|Privacy policy|Modern Slavery Act Transparency Statement|Sitemap|About US| Contact US: help@patsnap.com