Multi-label classifier constructing method based on cost-sensitive active learning
An active learning, cost-sensitive technology, applied in the field of multi-label classification, it can solve the problems of increasing the number of iterations, reducing the learning efficiency, and high cost of labeling samples, and achieving the effect of improving efficiency, reducing cost, and improving robustness.
- Summary
- Abstract
- Description
- Claims
- Application Information
AI Technical Summary
Problems solved by technology
Method used
Image
Examples
Embodiment 1
[0037] Embodiment 1: A method for constructing a multi-label classifier based on cost-sensitive active learning, including the following:
[0038] This example uses the Diagnosis data set, which has 3 labels: Cold, LungCancer, and Cough, and 258 samples. This example uses 30 samples, and each sample has 3 labels, that is, 90 sample-label pairs as marked The sample set L, the remaining 158 samples as the unlabeled set U, and 70 samples as the test set. The number of sample-label pairs selected each time is 3.
[0039] The misclassification cost of each label is set according to prior knowledge, as shown in the following table:
[0040] Cold Lung Cancer Cough C 11 0 0 0 C 10 5 50 7 C 01 1 1 1 C 00 0 0 0
[0041] In this embodiment, BRkNN is used as the basic classifier, and the initial classifier model is trained on the labeled set L , as the current classifier.
[0042] (1) Use the current classifier model to predict and...
Embodiment 2
[0056] Embodiment two: see figure 1 and image 3 As shown, a multi-label classifier construction method based on cost-sensitive active learning includes the following:
[0057] This embodiment adopts the flags data set, which has 7 labels and 194 samples in total, of which 135 samples are used to establish a pool and 59 samples are used for testing. 210 sample-label pairs are randomly selected to train the initial classifier, and 35 labels are selected for each iteration.
[0058] In this embodiment, BRkNN is used as the initial classifier algorithm to construct the initial classifier; the initial classifier is trained using the sample pool to obtain the current classifier;
[0059] Use the current classifier to classify the test samples, obtain the predicted label value, calculate the expected misclassification cost of the sample-label pair, select 35 highest-risk sample-label pairs to mark, add to the training set, retrain the classifier, and obtain an update After the c...
Embodiment 3
[0068] On the six data sets birds, enron, genbase, medical, CAL500 and bibtex shown in the table below, the method of the present invention is compared and verified.
[0069] The methods for comparison are:
[0070] LCam: the label-based cost-sensitive active learning method of the present invention;
[0071] ECam: a sample-based cost-sensitive active learning method;
[0072] ERnd: sample-based random selection active learning method;
[0073] LRnd: A Label-Based Random Selection Active Learning Approach.
[0074] Table 1 Dataset Properties
[0075] name field Number of samples Number of tags birds audio 322 19 enron text 1702 53 genbase biology 662 27 medical text 978 45 CAL500 music 502 174 bibtex text 7395 159
[0076] Table 2 is at the cost ratio of C 01 =1, C 10 When =2, the number of iterations required for cost-sensitive multi-label active learning methods based on samples and...
PUM
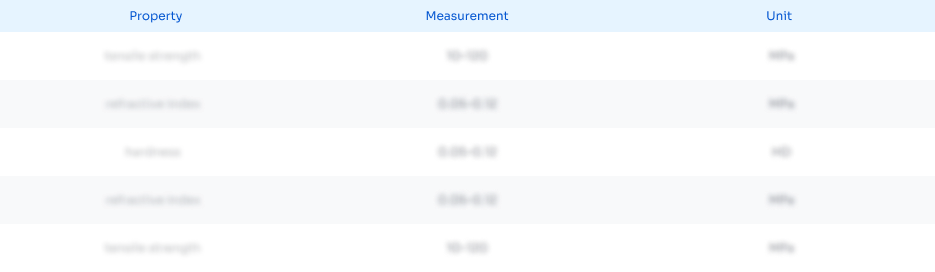
Abstract
Description
Claims
Application Information
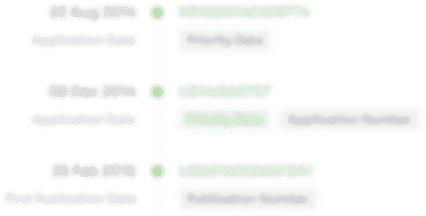
- R&D Engineer
- R&D Manager
- IP Professional
- Industry Leading Data Capabilities
- Powerful AI technology
- Patent DNA Extraction
Browse by: Latest US Patents, China's latest patents, Technical Efficacy Thesaurus, Application Domain, Technology Topic.
© 2024 PatSnap. All rights reserved.Legal|Privacy policy|Modern Slavery Act Transparency Statement|Sitemap