Understanding method of non-parametric RGB-D scene based on probabilistic graphical model
A probabilistic graphical model, RGB-D technology, applied in the field of non-parametric RGB-D scene understanding
- Summary
- Abstract
- Description
- Claims
- Application Information
AI Technical Summary
Problems solved by technology
Method used
Image
Examples
Embodiment 1
[0079] Image to be detected image 3 Part (a) is processed by the Superparsing (RGB) algorithm and the method of the present invention before and after combining the depth information. It can be seen from the result comparison chart that our method effectively overcomes the problem of insufficient color information on the labeling results under dim light conditions. Influence, achieve a good scene understanding effect.
Embodiment 2
[0081] Image to be detected Figure 4 Part (a) is processed by the Superparsing (RGB) algorithm and the method of the present invention before and after combining the depth information. It can be seen from the result comparison chart that our method effectively reduces the false matching when the colors of different types of objects are similar. After adding depth information, our method effectively overcomes the impact of insufficient color information on the labeling results under insufficient lighting conditions, and achieves a good scene understanding effect.
Embodiment 3
[0083] Image to be detected Figure 5 Part (a) is processed by the Superparsing (RGB) algorithm and the method of the present invention before and after combining the depth information. It can be seen from the result comparison chart that after adding the depth information, our method effectively reduces the number of objects in different categories with similar colors. Mis-matching, achieving a good scene understanding effect.
PUM
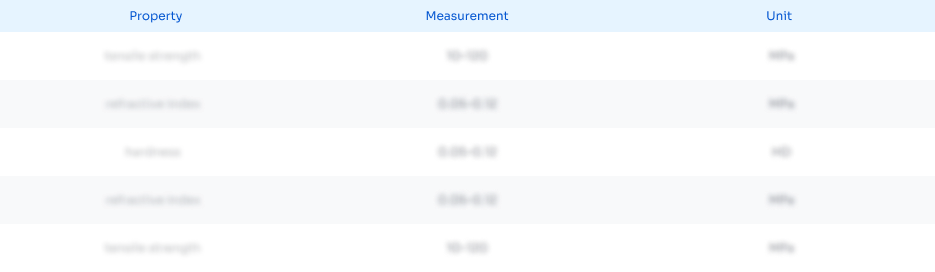
Abstract
Description
Claims
Application Information
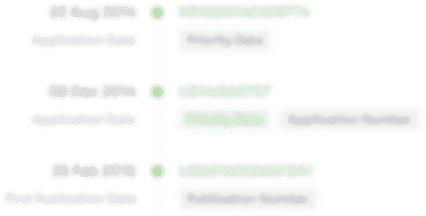
- R&D Engineer
- R&D Manager
- IP Professional
- Industry Leading Data Capabilities
- Powerful AI technology
- Patent DNA Extraction
Browse by: Latest US Patents, China's latest patents, Technical Efficacy Thesaurus, Application Domain, Technology Topic, Popular Technical Reports.
© 2024 PatSnap. All rights reserved.Legal|Privacy policy|Modern Slavery Act Transparency Statement|Sitemap|About US| Contact US: help@patsnap.com