Target matching method for multi-camera system based on deep convolutional neural network
A neural network, multi-camera technology, applied in the field of target matching based on deep convolutional neural network, can solve the problems of camera calibration difficulties, affecting the accuracy of matching results, and extraction problems.
- Summary
- Abstract
- Description
- Claims
- Application Information
AI Technical Summary
Problems solved by technology
Method used
Image
Examples
Embodiment Construction
[0024] The method of the invention includes two parts: extraction of target features and classification and identification of targets. Among them, the feature extraction of the target adopts the deep learning method. By constructing a multi-hidden layer neural network, the sample features are transformed layer by layer, and the feature representation of the sample in the original space is transformed into a new feature space to learn more useful features. Then use this feature as the input feature of the multi-class SVM classifier to classify and identify the target, so as to finally improve the accuracy of classification or prediction. attached figure 1 The implementation block diagram of this algorithm is shown, and the specific steps are as follows:
[0025] (1) Preprocessing of the target image: extract n target images in the multi-camera domain and divide them into m labels; use the bicubic interpolation algorithm (bicubic interpolation) to uniformly adjust the image siz...
PUM
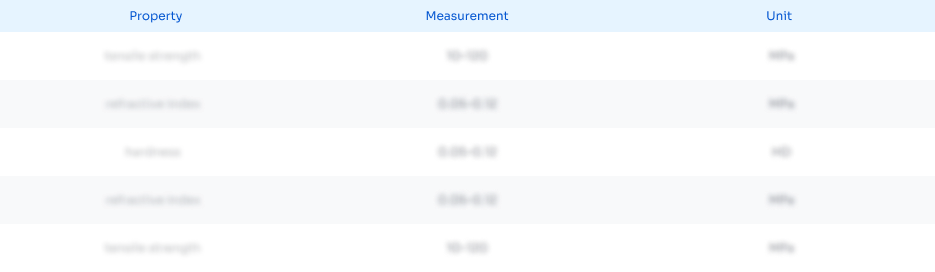
Abstract
Description
Claims
Application Information
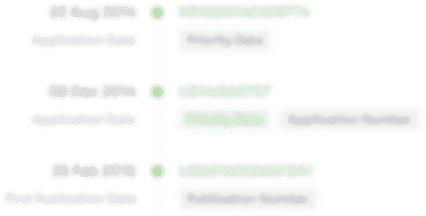
- R&D Engineer
- R&D Manager
- IP Professional
- Industry Leading Data Capabilities
- Powerful AI technology
- Patent DNA Extraction
Browse by: Latest US Patents, China's latest patents, Technical Efficacy Thesaurus, Application Domain, Technology Topic, Popular Technical Reports.
© 2024 PatSnap. All rights reserved.Legal|Privacy policy|Modern Slavery Act Transparency Statement|Sitemap|About US| Contact US: help@patsnap.com