City short-term water consumption prediction method based on least square support vector machine model
A technology of support vector machine and least squares, applied in forecasting, data processing applications, instruments, etc., can solve problems such as poor forecasting effect, large training error, falling into local extremum, etc., to improve accuracy, good generalization ability, Improving the performance of search capabilities
- Summary
- Abstract
- Description
- Claims
- Application Information
AI Technical Summary
Problems solved by technology
Method used
Image
Examples
Embodiment Construction
[0046] The conception, specific structure and technical effects of the present invention will be further described below in conjunction with the accompanying drawings, so as to fully understand the purpose, characteristics and effects of the present invention, but the protection of the present invention is not limited thereto.
[0047] The urban short-term water consumption prediction method based on the least squares support vector machine model of an embodiment of the present invention comprises the following steps:
[0048] (1) Obtain raw data, which includes historical water consumption series;
[0049] (2) Preprocessing the historical water consumption series to remove abnormal data in the historical water consumption series;
[0050] (3) Carry out correlation analysis to the historical water consumption sequence after pretreatment;
[0051] (4) Using the least squares support vector machine method to establish a short-term urban water consumption prediction model, select ...
PUM
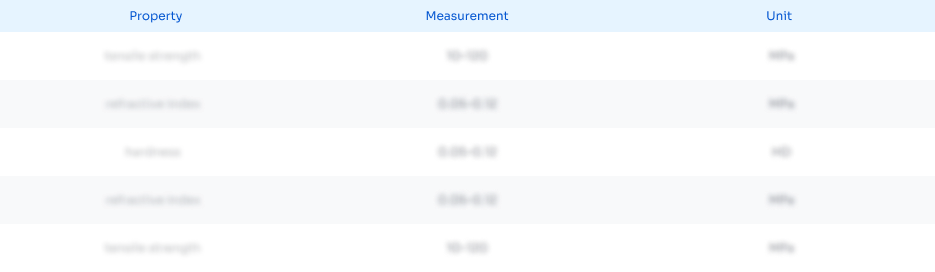
Abstract
Description
Claims
Application Information
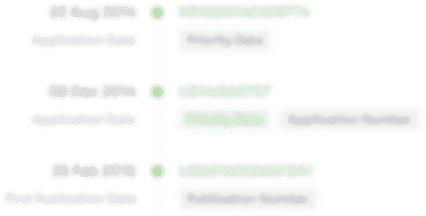
- R&D Engineer
- R&D Manager
- IP Professional
- Industry Leading Data Capabilities
- Powerful AI technology
- Patent DNA Extraction
Browse by: Latest US Patents, China's latest patents, Technical Efficacy Thesaurus, Application Domain, Technology Topic, Popular Technical Reports.
© 2024 PatSnap. All rights reserved.Legal|Privacy policy|Modern Slavery Act Transparency Statement|Sitemap|About US| Contact US: help@patsnap.com