A Supervised Multi-View Feature Selection Method Based on Automatic View Generation and Joint L1,2 Norm Minimization
A feature selection and multi-view technology, applied in character and pattern recognition, instruments, computing, etc., can solve the problems of high-resolution remote sensing image information loss, reduce feature redundancy, overcome information loss, and improve overall performance Effect
- Summary
- Abstract
- Description
- Claims
- Application Information
AI Technical Summary
Problems solved by technology
Method used
Image
Examples
specific Embodiment approach 1
[0065] Specific implementation mode one: the following combination figure 1 and figure 2 Describe this embodiment, which is based on automatic view generation and combination1 1,2 A supervised multi-view feature selection method for norm minimization, which includes the following steps:
[0066] Step 1: collect remote sensing image data, preprocess the remote sensing image data; then perform feature extraction to obtain a feature vector set; then normalize all feature vectors in the feature vector set to obtain the original feature vector set;
[0067] Step 2: Use the affinity propagation algorithm to generate feature multi-views from the original feature vector set obtained in step 1;
[0068] Step 3: Based on l 1,2 Norm performs supervised multi-view feature selection on the feature multi-view generated in step two.
[0069] This implementation is divided into three steps, one is data preparation; the other is view generation; the third is based on l 1,2 Feature select...
specific Embodiment approach 2
[0070] Specific implementation mode two: the following combination figure 1 and figure 2 Describe this embodiment. This embodiment will further explain Embodiment 1. The preprocessing of remote sensing image data in step 1 includes sequentially performing geometric fine correction and image registration, image mosaic and cropping, atmospheric correction and Bad tape removal.
[0071] This embodiment mainly includes bad band removal and data deformation and reorganization. Preprocessing is a very important step in remote sensing applications.
specific Embodiment approach 3
[0072] Specific implementation mode three: the following combination figure 1 and figure 2 Describe this embodiment mode. This embodiment mode will further explain Embodiment 1 or 2. The specific method for obtaining the feature vector set described in step 1 is: extracting the feature values of the preprocessed remote sensing image data to obtain feature data, and all feature data From n samples x in the m-dimensional feature space i Composition, characteristic data recorded as which sample the y i is x i mark, y i ∈{1,...,c}, c is the number of categories; put n samples x i The row vector of is denoted as the sample set X: The label vector corresponding to the sample set X is y, and the n samples x i The m eigenvectors formed by the column vectors are denoted as X=[f 1 ,f 2 ,...,f m ],and
[0073] The method to obtain the original feature vector set in step 1 is: the feature data All the eigenvalues in are mapped to [0-1] to obtain the original set of...
PUM
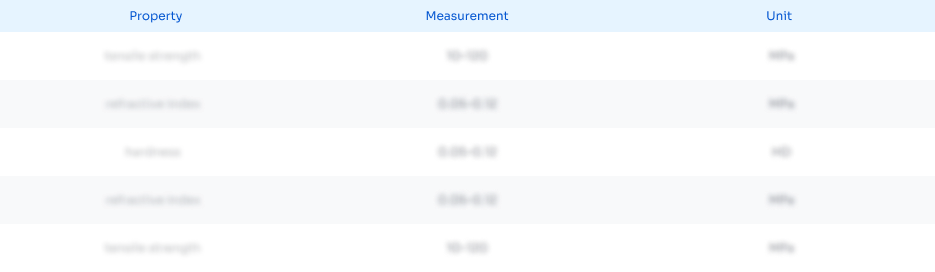
Abstract
Description
Claims
Application Information
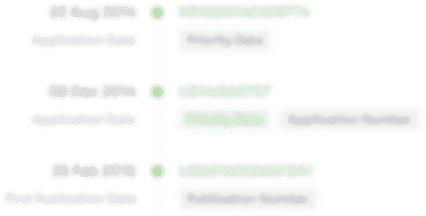
- R&D
- Intellectual Property
- Life Sciences
- Materials
- Tech Scout
- Unparalleled Data Quality
- Higher Quality Content
- 60% Fewer Hallucinations
Browse by: Latest US Patents, China's latest patents, Technical Efficacy Thesaurus, Application Domain, Technology Topic, Popular Technical Reports.
© 2025 PatSnap. All rights reserved.Legal|Privacy policy|Modern Slavery Act Transparency Statement|Sitemap|About US| Contact US: help@patsnap.com