Human gesture recognizing method based on depth convolution condition random field
A technology of human action recognition and conditional random field, applied in character and pattern recognition, computer components, instruments, etc., can solve problems such as inability to model image sequence data in space-time transformation, inability to model nonlinear relationships, and inability to predict
- Summary
- Abstract
- Description
- Claims
- Application Information
AI Technical Summary
Problems solved by technology
Method used
Image
Examples
Embodiment 1
[0029] 101: Alternately obtain the spatial feature expression of the input image sequence according to the spatial convolution layer and the sub-sampling layer;
[0030] 102: Perform temporal convolution operation on the spatial feature expression of the input image sequence to obtain further image sequence feature expression;
[0031] 103: Construct and optimize a deep conditional random field recognition model based on spatio-temporal convolutional network;
[0032] 104: Perform forward calculation of the depth-conditional random field recognition after optimizing the video sequence to be predicted, and obtain the action category label information to which each frame of the image in the video sequence to be predicted belongs.
[0033] Wherein, the depth conditional random field identification model in step 103 includes:
[0034] A state function for obtaining the relationship between the image data in the sequence and the category label after the nonlinear transformation; ...
Embodiment 2
[0043] The following combines calculation formulas, examples and figure 2 The scheme in Example 1 is described in detail, wherein the entire space-time convolutional network has two different operations, namely spatial convolution and temporal convolution, which will be described in detail below:
[0044] 201: Alternately obtain the spatial feature expression of the input image sequence according to the spatial convolution layer and the sub-sampling layer;
[0045] Among them, the spatial convolutional network mainly consists of alternating spatial convolutional layers and sub-sampling layers. The spatial convolution layer mainly detects the features in the input image, and the sub-sampling layer performs local averaging or local maximization operations to reduce image resolution and improve feature robustness. The main operations of the spatial convolution layer are expressed as follows:
[0046] The spatial convolution operation is to perform a convolution operation on th...
Embodiment 3
[0096] The feasibility of this method is verified by specific experiments below. The present invention uses two types of data sets to verify the proposed algorithm. One is a segmented action dataset that contains only one action in each video, and the other is a non-action-segmented dataset that contains multiple actions in each video. The two datasets and experimental results are described below.
[0097] see image 3 , the segmented Weizmann dataset is one of the commonly used standard datasets in action recognition tasks. This dataset contains 83 videos recorded by 9 individuals. There are 9 types of movements, namely running, walking, jumping jacks, jumping with two legs forward, jumping with both legs in place, bowing, waving with both hands, waving with one hand, and sliding. This method performs background clipping for each frame and centers the action. After preliminary processing, the size of the image is 103×129, and there are still a lot of blank areas on the ed...
PUM
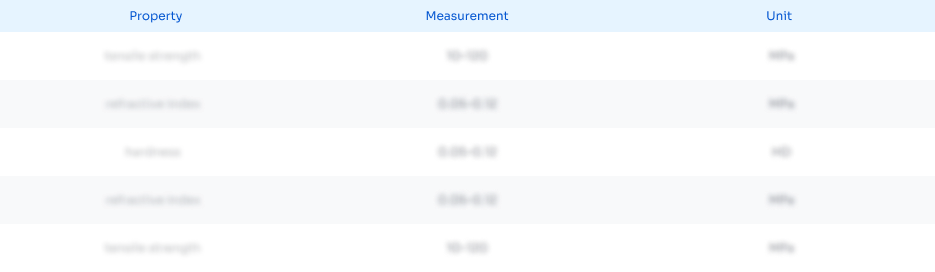
Abstract
Description
Claims
Application Information
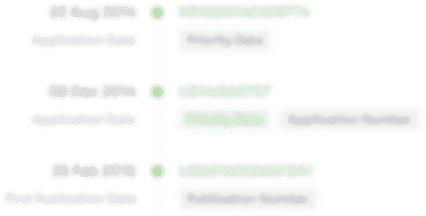
- R&D Engineer
- R&D Manager
- IP Professional
- Industry Leading Data Capabilities
- Powerful AI technology
- Patent DNA Extraction
Browse by: Latest US Patents, China's latest patents, Technical Efficacy Thesaurus, Application Domain, Technology Topic, Popular Technical Reports.
© 2024 PatSnap. All rights reserved.Legal|Privacy policy|Modern Slavery Act Transparency Statement|Sitemap|About US| Contact US: help@patsnap.com