Fabric defect classification method based on improved extreme learning machine
An extremely fast learning machine and classification method technology, which is applied in the field of fabric defect classification based on an improved extremely fast learning machine, can solve the problems of poor generalization ability and large training error, and achieves reduction of dependencies, improvement of generalization ability, and realization of The effect of real-time accurate detection
- Summary
- Abstract
- Description
- Claims
- Application Information
AI Technical Summary
Problems solved by technology
Method used
Image
Examples
Embodiment Construction
[0018] Below in conjunction with specific embodiment, further illustrate the present invention. It should be understood that these examples are only used to illustrate the present invention and are not intended to limit the scope of the present invention. In addition, it should be understood that after reading the teachings of the present invention, those skilled in the art can make various changes or modifications to the present invention, and these equivalent forms also fall within the scope defined by the appended claims of the present application.
[0019] In order to verify the effectiveness of the present invention, the present embodiment detects defects of 8 kinds of plain weave defect images: slub, broken warp, oil stain, weft broken, missing weft, missing warp, hanging warp, and weft shrinkage. There are 50 pictures for each defect, 20 pictures are used as training, and 30 pictures are used as test samples, and compared with ELM, OS-ELM, SAOS-ELM to verify the perform...
PUM
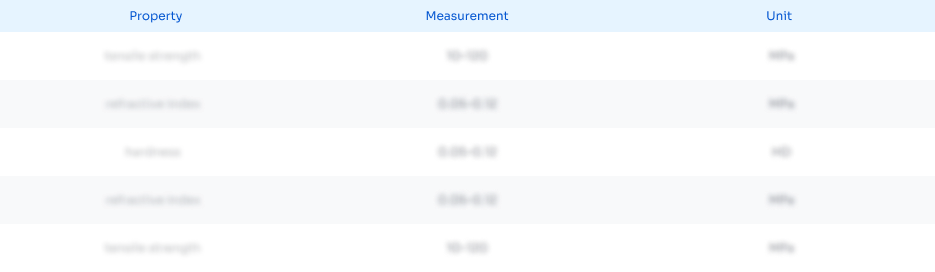
Abstract
Description
Claims
Application Information
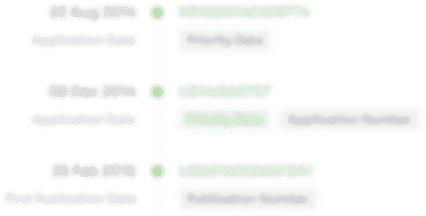
- R&D Engineer
- R&D Manager
- IP Professional
- Industry Leading Data Capabilities
- Powerful AI technology
- Patent DNA Extraction
Browse by: Latest US Patents, China's latest patents, Technical Efficacy Thesaurus, Application Domain, Technology Topic, Popular Technical Reports.
© 2024 PatSnap. All rights reserved.Legal|Privacy policy|Modern Slavery Act Transparency Statement|Sitemap|About US| Contact US: help@patsnap.com