Significant guidance and unsupervised feature learning based image classification method
A feature learning and classification method technology, applied in the direction of instruments, character and pattern recognition, computer components, etc., can solve problems such as defects and inappropriateness, and achieve the effects of preventing overfitting, improving classification accuracy, and reducing classification time consumption
- Summary
- Abstract
- Description
- Claims
- Application Information
AI Technical Summary
Problems solved by technology
Method used
Image
Examples
Embodiment Construction
[0048] The present invention will be further described below in conjunction with the accompanying drawings. The following examples are only used to illustrate the technical solution of the present invention more clearly, but not to limit the protection scope of the present invention.
[0049] This embodiment takes the STL-10 database as an example, the database includes 10 types of RGB images, and the size of each image is 96*96. Among them, the number of unlabeled images for unsupervised training is 100,000, the number of training samples for supervised training is 5,000, and the number of testing samples is 8,000.
[0050] In conjunction with the steps of the present invention, the specific process is as follows:
[0051] 1.1) Using a context-aware saliency detection algorithm, compute a saliency map for each image in the unlabeled data sample.
[0052] 1.2) Arrange the pixels in each saliency map in descending order according to the size of the gray value.
[0053] 1.3) ...
PUM
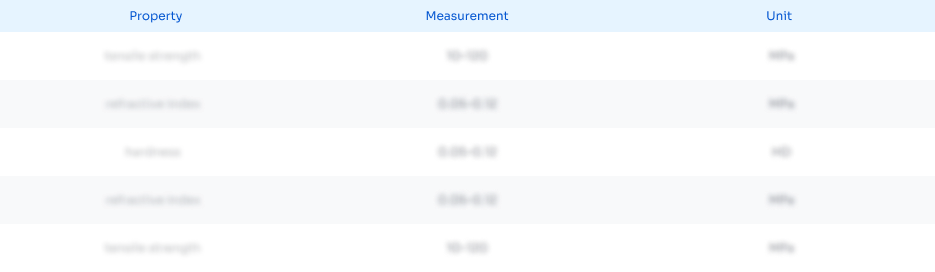
Abstract
Description
Claims
Application Information
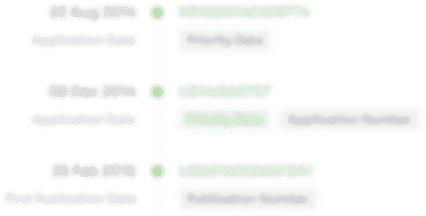
- R&D
- Intellectual Property
- Life Sciences
- Materials
- Tech Scout
- Unparalleled Data Quality
- Higher Quality Content
- 60% Fewer Hallucinations
Browse by: Latest US Patents, China's latest patents, Technical Efficacy Thesaurus, Application Domain, Technology Topic, Popular Technical Reports.
© 2025 PatSnap. All rights reserved.Legal|Privacy policy|Modern Slavery Act Transparency Statement|Sitemap|About US| Contact US: help@patsnap.com