Pedestrian image detection method based on sparse coding and neural network
A neural network, sparse coding technology, applied in the field of image retrieval, can solve the problem of difficult training of Adboost+SVM model
- Summary
- Abstract
- Description
- Claims
- Application Information
AI Technical Summary
Problems solved by technology
Method used
Image
Examples
Embodiment Construction
[0061] The embodiment of the present invention provides a pedestrian picture detection method based on sparse coding and neural network, such as figure 1 As shown, specifically:
[0062] Step S101: First, image preprocessing is performed on the positive samples and negative samples in the sample data set, and then the aggregated channel features are extracted; the sample data set is the INRIA training sample set and the symmetrical transformation sample set of the INRIA training sample set, and the INRIA training sample set is At present, the most used static pedestrian database has higher definition and can train more accurate classifiers. Among them, the images of positive samples contain pedestrians, and the images of negative samples do not contain pedestrians;
[0063] Step S102: Construct a BP-AdaBoost strong classifier model, and train the BP-AdaBoost strong classifier using the aggregation channel features obtained in step S101;
[0064] Step S103: Obtain the video fr...
PUM
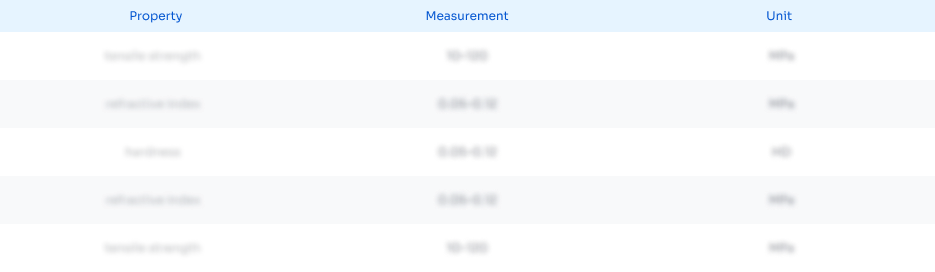
Abstract
Description
Claims
Application Information
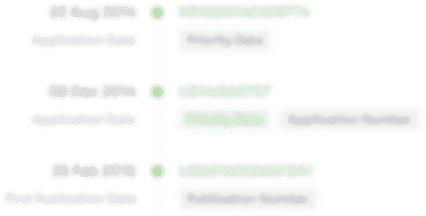
- Generate Ideas
- Intellectual Property
- Life Sciences
- Materials
- Tech Scout
- Unparalleled Data Quality
- Higher Quality Content
- 60% Fewer Hallucinations
Browse by: Latest US Patents, China's latest patents, Technical Efficacy Thesaurus, Application Domain, Technology Topic, Popular Technical Reports.
© 2025 PatSnap. All rights reserved.Legal|Privacy policy|Modern Slavery Act Transparency Statement|Sitemap|About US| Contact US: help@patsnap.com