Image multi-tag marking algorithm based on multi-example package feature learning
A feature learning, multi-example technology, applied in image enhancement, image analysis, image data processing and other directions, can solve problems such as being unable to adapt to actual needs
- Summary
- Abstract
- Description
- Claims
- Application Information
AI Technical Summary
Problems solved by technology
Method used
Image
Examples
Embodiment Construction
[0059] The present invention is described in detail below:
[0060] An image multi-label labeling algorithm based on multi-instance bag feature learning, the method specifically includes the following steps:
[0061] Step 1: Obtain the training image set and segment the images in it to obtain the block image set of all images; the image segmentation algorithm uses the pixel RGB color value as the clustering target, and uses the fuzzy C-means clustering (FCM) algorithm for image segmentation .
[0062] Step 2: Extract color histogram features and orientation gradient histogram features (HOG features) for each image block in the training set. Specific steps are as follows:
[0063] Step 2.1: Extract the R, G, and B color values of each pixel of the image block respectively;
[0064] Step 2.2: Divide the color values into 16 groups on average, and use 16 as the group distance to count the number of pixels of R, G, and B three color values in each group of color value ran...
PUM
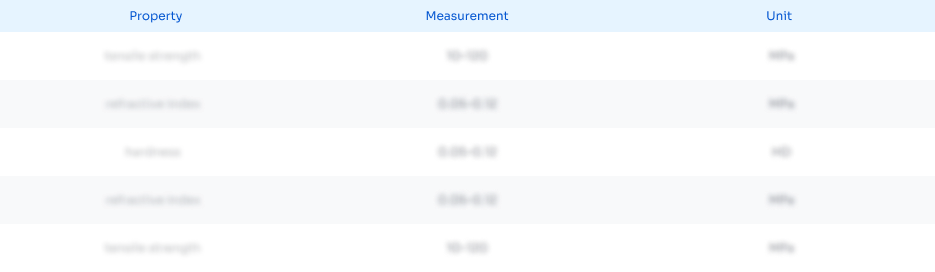
Abstract
Description
Claims
Application Information
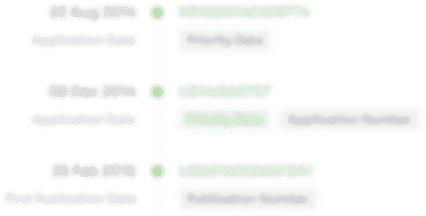
- R&D Engineer
- R&D Manager
- IP Professional
- Industry Leading Data Capabilities
- Powerful AI technology
- Patent DNA Extraction
Browse by: Latest US Patents, China's latest patents, Technical Efficacy Thesaurus, Application Domain, Technology Topic, Popular Technical Reports.
© 2024 PatSnap. All rights reserved.Legal|Privacy policy|Modern Slavery Act Transparency Statement|Sitemap|About US| Contact US: help@patsnap.com