Image visual attribute excavation method based on sparse factor analysis
A technology of image vision and sparse factor, applied in the field of image analysis, it can solve the problems of semantic deviation and low efficiency of manual labeling process, and achieve the effect of improving the distinguishing performance.
- Summary
- Abstract
- Description
- Claims
- Application Information
AI Technical Summary
Problems solved by technology
Method used
Image
Examples
Embodiment Construction
[0023] The image visual attribute mining method based on sparse factor analysis provided by the present invention will be described in detail below in conjunction with the accompanying drawings.
[0024] figure 1 It is a schematic flow chart of the present invention. As shown in the figure, it includes two steps: the first step is to calculate the feature matrix of the image collection, and the second step is to mine the visual attributes of the image collection.
[0025] figure 2 In order to use the visual attribute results mined from 435 face images in the Caltech101 dataset, the first step is to extract the gradient histogram features for each image (the feature extraction parameters are: the image is decomposed into 8 pixels × 8 pixels of cells, in each The gradient direction histogram of 0°-180° is counted in the cell, and then normalized by 2 cell × 2 cell blocks, a descriptor (or feature vector) is generated for each cell, and finally all the cells are concatenated ...
PUM
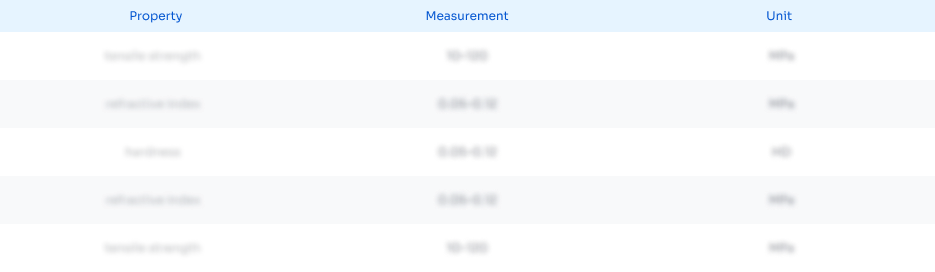
Abstract
Description
Claims
Application Information
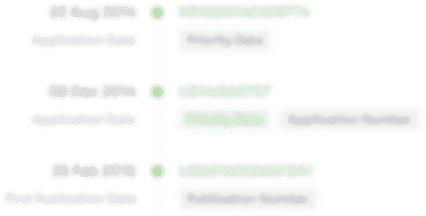
- R&D Engineer
- R&D Manager
- IP Professional
- Industry Leading Data Capabilities
- Powerful AI technology
- Patent DNA Extraction
Browse by: Latest US Patents, China's latest patents, Technical Efficacy Thesaurus, Application Domain, Technology Topic.
© 2024 PatSnap. All rights reserved.Legal|Privacy policy|Modern Slavery Act Transparency Statement|Sitemap