Cross-modal hash retrieval algorithm based on fine-grained similarity matrix
A similarity matrix and hash algorithm technology, applied in the field of cross-modal retrieval, can solve the problem that the cross-modal hash algorithm cannot mine the similarity information of data items, and achieve the effect of avoiding quantization errors.
- Summary
- Abstract
- Description
- Claims
- Application Information
AI Technical Summary
Problems solved by technology
Method used
Image
Examples
Embodiment Construction
[0060] It can be known from the background art that the existing two-stage cross-modal hashing algorithm has two main defects. First, the current two-stage hashing algorithms all use coarse-grained similarity matrices, which cannot mine the rich similarity information of data items in the original space. Second, most two-stage hash algorithms use multi-classification to train hash codes, which may not get the best hash function. Therefore, in this embodiment, for the above two problems, the similarity matrix in the fine-grained definition mode is respectively used, and the training mode of the hash function is redesigned to solve the above two problems.
[0061] In this embodiment, in the hash function learning in the second stage, for the image mode, the CNN-F network pre-trained on ImageNet is used. Keep the first five convolutional layers convl~conv5 and the next two fully connected layers fc6~fc7 unchanged, and replace the eighth fully connected layer with a new fully con...
PUM
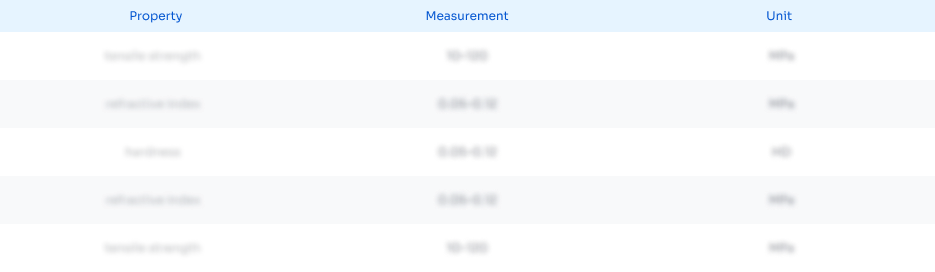
Abstract
Description
Claims
Application Information
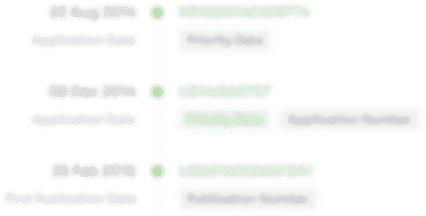
- R&D Engineer
- R&D Manager
- IP Professional
- Industry Leading Data Capabilities
- Powerful AI technology
- Patent DNA Extraction
Browse by: Latest US Patents, China's latest patents, Technical Efficacy Thesaurus, Application Domain, Technology Topic.
© 2024 PatSnap. All rights reserved.Legal|Privacy policy|Modern Slavery Act Transparency Statement|Sitemap