Electronic nose gas identification method based on source domain transfer limit learning drift compensation
An extreme learning and drift compensation technology, applied in scientific instruments, instruments, measuring devices, etc., can solve problems such as improving the gas recognition accuracy of electronic noses, difficulty in drift compensation, and the inability of the recognition neural network to correctly identify matching gases.
- Summary
- Abstract
- Description
- Claims
- Application Information
AI Technical Summary
Problems solved by technology
Method used
Image
Examples
Embodiment Construction
[0068] Aiming at the problem that the gas sensor drift of the electronic nose affects the gas identification accuracy, the present invention provides an electronic nose gas identification method based on source domain migration limit learning drift compensation, and analyzes and solves the problem from the perspective of a machine learning machine , a concept based on source domain migration limit learning is proposed, and the source domain data set and the target domain data set are respectively constructed with the help of a small number of electronic noses with the tagged gas sensor array sensing data matrix collected without drifting and drifting , used for source domain migration limit learning to obtain a robust identification classifier, which can improve the tolerance performance of the identification classifier for gas identification after the electronic nose drifts, and then use the identification classifier obtained after learning to perform When identifying the gas ...
PUM
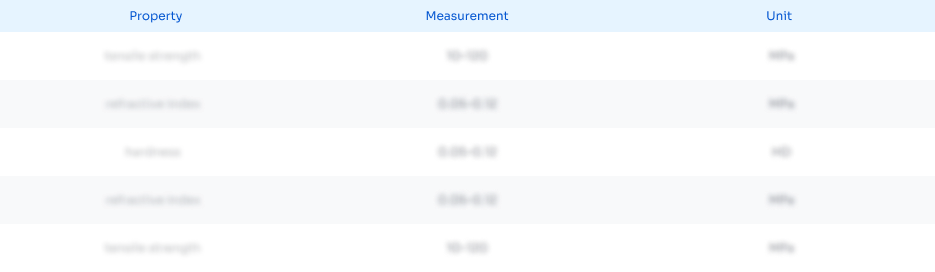
Abstract
Description
Claims
Application Information
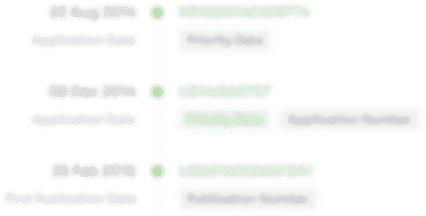
- R&D Engineer
- R&D Manager
- IP Professional
- Industry Leading Data Capabilities
- Powerful AI technology
- Patent DNA Extraction
Browse by: Latest US Patents, China's latest patents, Technical Efficacy Thesaurus, Application Domain, Technology Topic, Popular Technical Reports.
© 2024 PatSnap. All rights reserved.Legal|Privacy policy|Modern Slavery Act Transparency Statement|Sitemap|About US| Contact US: help@patsnap.com