A Universal Calibration Method for Electronic Nose Drift Based on Convex Set Projection and Extreme Learning Machine
An extreme learning machine and convex set projection technology, applied in scientific instruments, computer parts, standard gas analyzers, etc., can solve the limitation of domain transfer ability and generalization, poor generalization, and interrupted eigenvalue distribution rules, etc. problem, to achieve good generalization and migration ability, improve gas recognition accuracy, and good scalability.
- Summary
- Abstract
- Description
- Claims
- Application Information
AI Technical Summary
Problems solved by technology
Method used
Image
Examples
Embodiment Construction
[0057] The embodiment of the present invention will be explained in detail below in conjunction with the accompanying drawings. The examples given are only for the purpose of illustration, and cannot be interpreted as limiting the present invention. The accompanying drawings are only for reference and description, and do not constitute the scope of patent protection of the present invention. limitations, since many changes may be made in the invention without departing from the spirit and scope of the invention.
[0058] In order to improve the gas recognition accuracy and generalization of the electronic nose after its gas sensor drifts, the embodiment of the present invention provides a general calibration method for electronic nose drift based on convex set projection and extreme learning machine, such as figure 1 , 4 shown, including steps:
[0059] S0. Collect the undrifted and drifted output responses of a sensor array composed of multiple sensors in a variety of standa...
PUM
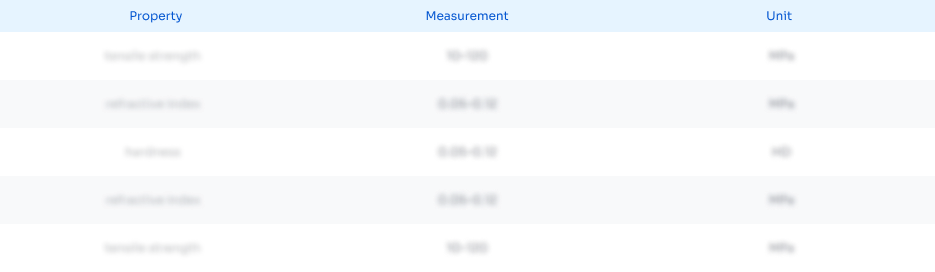
Abstract
Description
Claims
Application Information
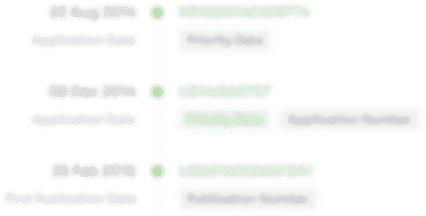
- R&D Engineer
- R&D Manager
- IP Professional
- Industry Leading Data Capabilities
- Powerful AI technology
- Patent DNA Extraction
Browse by: Latest US Patents, China's latest patents, Technical Efficacy Thesaurus, Application Domain, Technology Topic, Popular Technical Reports.
© 2024 PatSnap. All rights reserved.Legal|Privacy policy|Modern Slavery Act Transparency Statement|Sitemap|About US| Contact US: help@patsnap.com