Distributed fast frequent item set mining method based on Apriori
A frequent item set mining and distributed technology, which is applied in special data processing applications, instruments, electrical digital data processing, etc., can solve problems such as memory leaks, achieve simplified statistics, meet the needs of frequent item set mining, and avoid frequent scanning Effect
- Summary
- Abstract
- Description
- Claims
- Application Information
AI Technical Summary
Problems solved by technology
Method used
Image
Examples
Embodiment Construction
[0014] Below in conjunction with specific embodiments, the present invention will be further illustrated, and it should be understood that these embodiments are only used to illustrate the present invention and not to limit the scope of the present invention. The modifications all fall within the scope defined by the appended claims of this application.
[0015] All Apriori-like association rule mining methods mainly include two main processes: frequent itemset generation and pruning. The distributed frequent itemset mining proposed in this paper is no exception. The main similarities and differences are mainly reflected in Spark-based parallelization. The implementation part is described in detail below. In terms of implementation, the whole method can be divided into preprocessing ( image 3 (a)) and iterative ( image 3 (b)) Two steps, both of which include candidate item set generation and pruning.
[0016] The method handles input datasets such as figure 1 shown, the ...
PUM
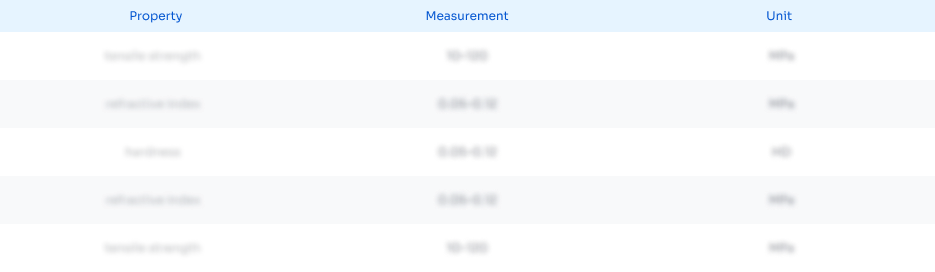
Abstract
Description
Claims
Application Information
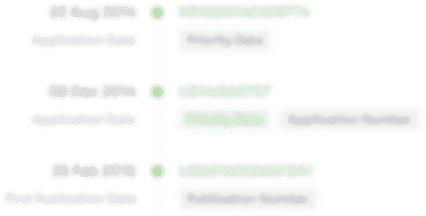
- R&D
- Intellectual Property
- Life Sciences
- Materials
- Tech Scout
- Unparalleled Data Quality
- Higher Quality Content
- 60% Fewer Hallucinations
Browse by: Latest US Patents, China's latest patents, Technical Efficacy Thesaurus, Application Domain, Technology Topic, Popular Technical Reports.
© 2025 PatSnap. All rights reserved.Legal|Privacy policy|Modern Slavery Act Transparency Statement|Sitemap|About US| Contact US: help@patsnap.com