Method for modeling and recognizing time sequence
A time series and sequence technology, applied in character and pattern recognition, instruments, computer parts, etc., can solve the problems of large data block dimensions, data distortion, and reduce the operation efficiency of later recognition algorithms, so as to improve recognition accuracy and reduce complexity. degree of effect
- Summary
- Abstract
- Description
- Claims
- Application Information
AI Technical Summary
Problems solved by technology
Method used
Image
Examples
Embodiment Construction
[0020] The present invention proposes a method for modeling and identifying time series, comprising the following steps:
[0021] 1) The dynamic data formed by arranging the data collected by the sensor according to the collection time sequence constitutes L (for example, 200) time series, and randomly selects 60%-80% of the time series (set as N) as the training set, and the remaining The time series below is used as the test set (the higher the percentage of the training set in the overall time series, the higher the recognition accuracy, which can be selected according to the specific operation accuracy requirements);
[0022] 2) Model each time series in the training set using a linear dynamic system model:
[0023] x ( t + 1 ) = A ...
PUM
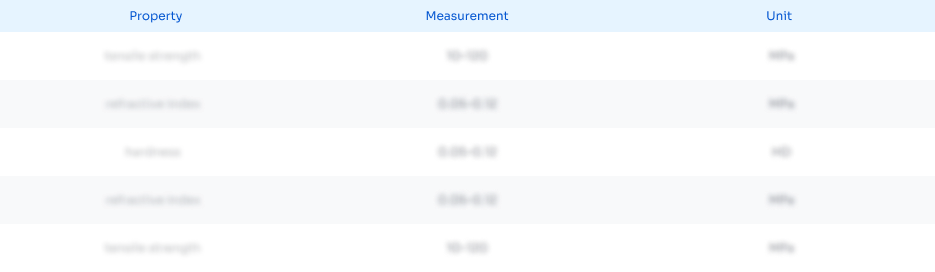
Abstract
Description
Claims
Application Information
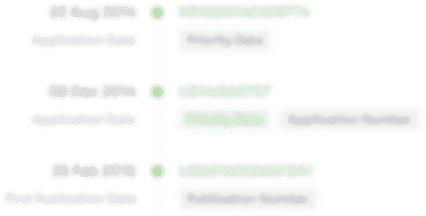
- R&D Engineer
- R&D Manager
- IP Professional
- Industry Leading Data Capabilities
- Powerful AI technology
- Patent DNA Extraction
Browse by: Latest US Patents, China's latest patents, Technical Efficacy Thesaurus, Application Domain, Technology Topic.
© 2024 PatSnap. All rights reserved.Legal|Privacy policy|Modern Slavery Act Transparency Statement|Sitemap