Multi-feature-fusion-convolutional-neural-network-based plankton image classification method
A convolutional neural network and multi-feature fusion technology, applied in the field of plankton image classification, can solve problems such as difficulty in classifying plankton images, and achieve the effect of improving the accuracy rate
- Summary
- Abstract
- Description
- Claims
- Application Information
AI Technical Summary
Problems solved by technology
Method used
Image
Examples
Embodiment
[0029] A plankton image classification method based on multi-feature fusion convolutional neural network, such as figure 1 shown, including the following steps:
[0030] S1: Collect clear plankton images and build a large-scale multi-category plankton image data set. The plankton images in this data set are used as original feature images. The number of collected images is about 30,000 to 90,000, and the types of plankton are About 30 to 50 categories;
[0031] S2: Process the original feature image, extract the global feature of plankton, and obtain the global feature image. The specific processing steps are:
[0032] S21: Using the image segmentation Scharr operator to convert the original feature image, the converted image includes global features and local features;
[0033] S22: Using a bilateral filtering method to remove local features in the converted image;
[0034] S23: Enhance the contrast to highlight the global features in the converted image;
[0035] S3: Pro...
PUM
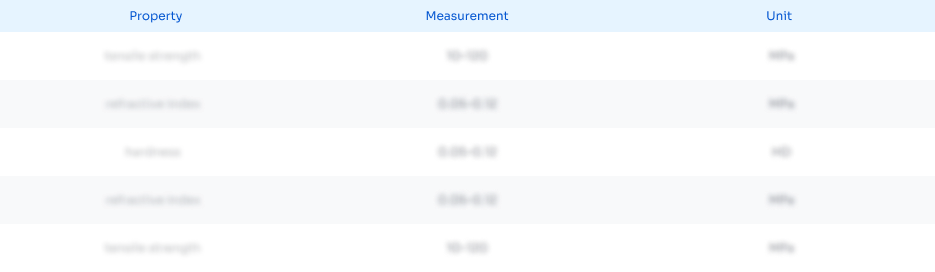
Abstract
Description
Claims
Application Information
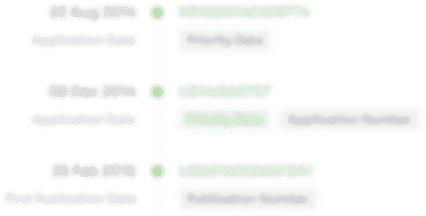
- R&D Engineer
- R&D Manager
- IP Professional
- Industry Leading Data Capabilities
- Powerful AI technology
- Patent DNA Extraction
Browse by: Latest US Patents, China's latest patents, Technical Efficacy Thesaurus, Application Domain, Technology Topic, Popular Technical Reports.
© 2024 PatSnap. All rights reserved.Legal|Privacy policy|Modern Slavery Act Transparency Statement|Sitemap|About US| Contact US: help@patsnap.com