Zero sample image classification method based on category attribute migration learning
A sample image, transfer learning technology, applied to computer parts, character and pattern recognition, instruments, etc., can solve the problem that the similarity cannot be directly measured, achieve the effect of simple and feasible practicality, and improve the classification accuracy
- Summary
- Abstract
- Description
- Claims
- Application Information
AI Technical Summary
Problems solved by technology
Method used
Image
Examples
Embodiment Construction
[0032] The invention relates to a category attribute migration learning technology for the field of zero-sample image classification. Aiming at the problem of obvious changes in the internal attributes of the category in the zero-sample image classification, it improves the traditional indirect attribute prediction method and achieves effective mining of visual samples. Deep semantic information, better prediction of attribute features of visual samples.
[0033] The purpose of the present invention is to provide a zero-shot image classification method based on category attribute transfer learning. At present, a commonly used idea in zero-shot learning is to transfer information by connecting labeled categories and unseen categories through attribute features, so as to obtain predicted semantic features of test samples. How to transfer information is one of the key technologies. Aiming at this key technology, the present invention proposes a learning framework that effectivel...
PUM
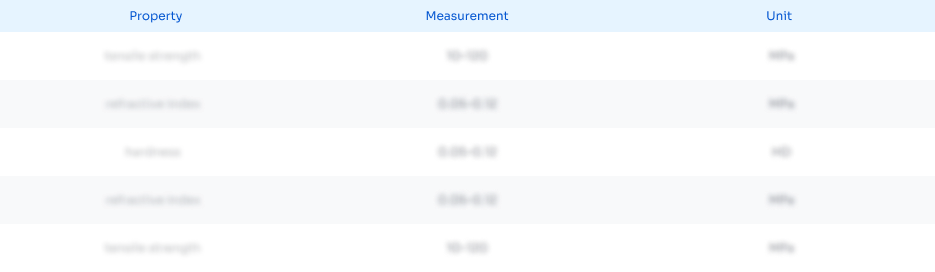
Abstract
Description
Claims
Application Information
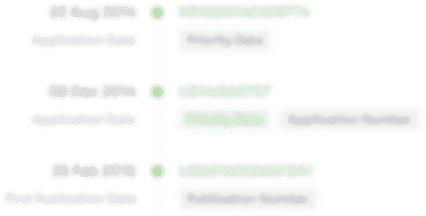
- Generate Ideas
- Intellectual Property
- Life Sciences
- Materials
- Tech Scout
- Unparalleled Data Quality
- Higher Quality Content
- 60% Fewer Hallucinations
Browse by: Latest US Patents, China's latest patents, Technical Efficacy Thesaurus, Application Domain, Technology Topic, Popular Technical Reports.
© 2025 PatSnap. All rights reserved.Legal|Privacy policy|Modern Slavery Act Transparency Statement|Sitemap|About US| Contact US: help@patsnap.com