Multi-sensor-based assessment system and method for students' classroom mastery
A mastery, multi-sensor technology, applied in the field of image and voice processing, can solve the problems of not considering the role of students, processing simplification, consuming large machine memory and computing time, etc.
- Summary
- Abstract
- Description
- Claims
- Application Information
AI Technical Summary
Problems solved by technology
Method used
Image
Examples
Embodiment 1
[0053] The present invention is a multi-sensor-based assessment system for students' classroom mastery, see figure 1 , including a video collection module, a facial expression classifier module, an audio collection module, a voice classifier module, a score entry module, a score classifier module, a decision fusion module, and a classroom teaching student reflection evaluation and suggestion module;
[0054] There are 3 information source modules, which are video collection module, audio collection module, and score entry module. The video collection module is connected to the facial expression classifier module to output facial expression classification results, and the audio collection module is connected to the voice classifier module to output voice classification. As a result, the score input module and the score classifier module are connected to output the score classification results. The above three classification results are all input into the decision-making fusion m...
Embodiment 2
[0065] The overall composition of the multi-sensor-based student classroom mastery evaluation system is the same as that in Embodiment 1. The classification confidence of the classifier is obtained based on the spatial distribution of samples described in the decision-making fusion module, specifically when the Gaussian mixture of each emotional category in the classifier When the likelihood of the model (GMM) is basically equal, it is considered that the sample is in the overlapping area of the probability distribution model, and the decision confidence of the classifier is low; when the likelihood values of the emotional categories given by the classifier are scattered, it is considered that The samples are in the non-overlapping region of the probability distribution model, and the decision confidence of the classifier is high.
[0066] The parameter in GMM is to use the training sample {x 1 ,x 2 ,...x m}, obtained by calculating the maximum likelihood estimation meth...
Embodiment 3
[0076] The overall composition of the student's classroom mastery evaluation system based on multiple sensors is the same as that of embodiment 1-2, wherein the voice classifier module first obtains the energy spectrum of the voice signal, and the energy spectrum of the voice signal passes through the Mel scale (Mel-scale) Triangular filter bank, calculate its logarithmic energy and normalize it, input it to the convolutional neural network for voice feature map classification, use the convolutional neural network (CNN), give the voice classification result, the result is expressed as irritability, joy and calm, as samples input to the decision fusion module.
[0077] In order to avoid complex artificial feature vector extraction operations in the speech emotion classification and recognition, the present invention selects Mel Frequency Spectrum Coefficients (MFCC), organically combines the auditory perception characteristics of the human ear with the generation mechanism of sp...
PUM
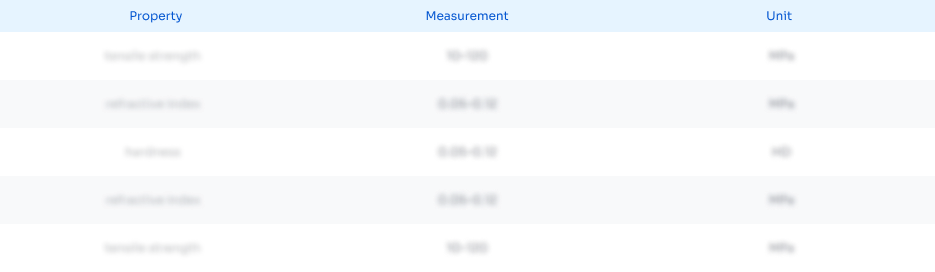
Abstract
Description
Claims
Application Information
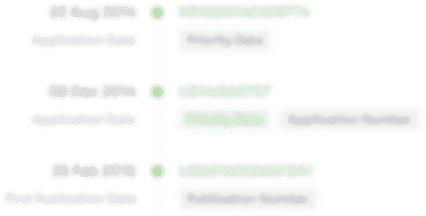
- Generate Ideas
- Intellectual Property
- Life Sciences
- Materials
- Tech Scout
- Unparalleled Data Quality
- Higher Quality Content
- 60% Fewer Hallucinations
Browse by: Latest US Patents, China's latest patents, Technical Efficacy Thesaurus, Application Domain, Technology Topic, Popular Technical Reports.
© 2025 PatSnap. All rights reserved.Legal|Privacy policy|Modern Slavery Act Transparency Statement|Sitemap|About US| Contact US: help@patsnap.com