Deep learning-based picture sentiment polarity analysis method
An emotion polarity, deep learning technology, applied in semantic analysis, special data processing applications, network data retrieval and other directions, can solve the problems of small data set, unsatisfactory final prediction accuracy, limited performance, etc., to achieve large data scale. Effect
- Summary
- Abstract
- Description
- Claims
- Application Information
AI Technical Summary
Problems solved by technology
Method used
Image
Examples
Embodiment Construction
[0078] In order to make the object, technical solution and advantages of the present invention clearer, the present invention will be further described in detail below in conjunction with the accompanying drawings. The purpose of the present invention is to provide a kind of picture emotion polarity analysis method based on deep learning, its frame is as follows figure 1 shown. The present invention will be further described in detail below in conjunction with the accompanying drawings and examples.
[0079] The realization steps of this invention are as follows:
[0080] 1. Data acquisition
[0081] This method can be applied to most image social networking sites with image search functions. In the specific implementation process, we chose Flickr, a photo social networking site, to collect data. For a retrieval request, Flickr currently allows a peak of 2000 images to be returned.
[0082] 1.1. Prior knowledge preparation
[0083] In the implementation process, we choos...
PUM
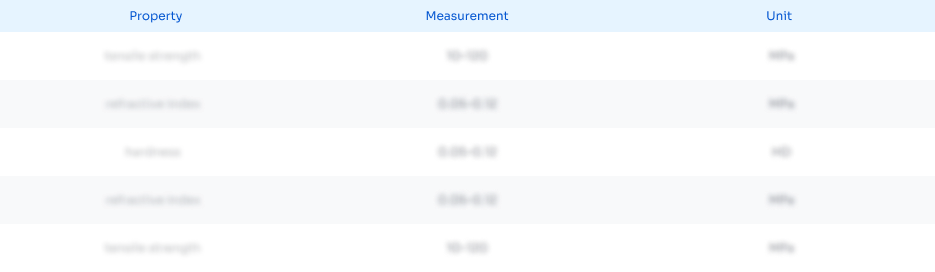
Abstract
Description
Claims
Application Information
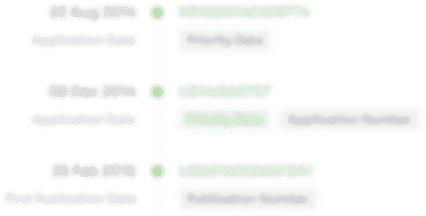
- Generate Ideas
- Intellectual Property
- Life Sciences
- Materials
- Tech Scout
- Unparalleled Data Quality
- Higher Quality Content
- 60% Fewer Hallucinations
Browse by: Latest US Patents, China's latest patents, Technical Efficacy Thesaurus, Application Domain, Technology Topic, Popular Technical Reports.
© 2025 PatSnap. All rights reserved.Legal|Privacy policy|Modern Slavery Act Transparency Statement|Sitemap|About US| Contact US: help@patsnap.com