Remote sensing image classification method based on AGA-PKF-SVM
An AGA-PKF-SVM, remote sensing image technology, applied in the field of remote sensing image processing, can solve the problems of poor generalization performance, inability to guarantee the classification effect, not fully considering the over-fitting of the support vector machine classification model, etc. The effect of fitting, good generalization performance
- Summary
- Abstract
- Description
- Claims
- Application Information
AI Technical Summary
Problems solved by technology
Method used
Image
Examples
Embodiment Construction
[0031] The specific implementation manners of the present invention will be further described in detail below in conjunction with the accompanying drawings and embodiments. The following examples are used to illustrate the present invention, but are not intended to limit the scope of the present invention.
[0032] like figure 1 Shown is the flow chart of the remote sensing image classification method based on AGA-PKF-SVM, and the specific method is as follows.
[0033] Step 1: The kernel function of the support vector machine adopts a polynomial kernel function, as shown in formula (1);
[0034]
[0035] Among them, K(x i , x j ) represents the kernel function, x i and x j Respectively represent two points in two-dimensional space, γ represents the inner product coefficient in the polynomial kernel function, r represents the constant term, and d represents the number of polynomial terms;
[0036] The penalty factor C in the support vector machine, the parameter γ in ...
PUM
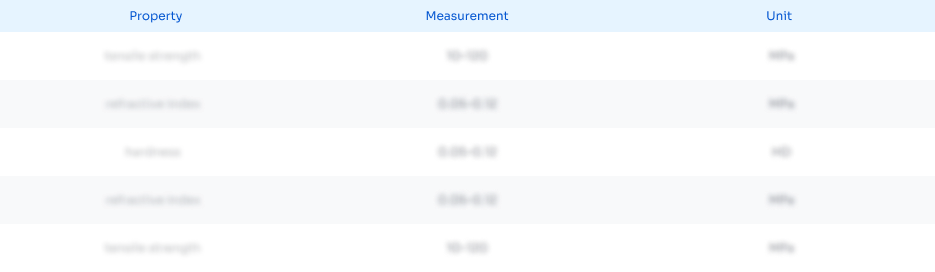
Abstract
Description
Claims
Application Information
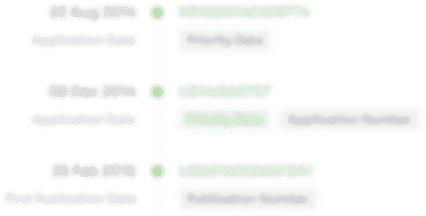
- R&D
- Intellectual Property
- Life Sciences
- Materials
- Tech Scout
- Unparalleled Data Quality
- Higher Quality Content
- 60% Fewer Hallucinations
Browse by: Latest US Patents, China's latest patents, Technical Efficacy Thesaurus, Application Domain, Technology Topic, Popular Technical Reports.
© 2025 PatSnap. All rights reserved.Legal|Privacy policy|Modern Slavery Act Transparency Statement|Sitemap|About US| Contact US: help@patsnap.com