Monte Carlo cross verification-based multiple-soft sensing algorithm cluster modeling method
A technology of cross-validation and modeling methods, which is applied in the field of multiple soft sensor algorithm cluster modeling to achieve the effect of improving accuracy
- Summary
- Abstract
- Description
- Claims
- Application Information
AI Technical Summary
Problems solved by technology
Method used
Image
Examples
Embodiment Construction
[0047] In order to overcome the outlier problem, the present invention systematically applies the model evaluation and outlier search aspects of the model cluster analysis method, and attempts to construct an integrated learning system under the framework of the model cluster analysis method. In terms of finding outliers, the Monte Carlo cross-validation algorithm MC based on the model cluster analysis method is used. After eliminating outliers, a variety of soft sensor algorithms are selected to model the industrial process, and then the prediction of each soft sensor algorithm is performed. The results are integrated. In terms of model evaluation, by generating a large number of training clusters, the influence of the selection of the training set on the model evaluation results is eliminated, and the diversity of data is improved.
[0048] The effectiveness of the present invention is illustrated below in conjunction with the example of a specific industrial process. In th...
PUM
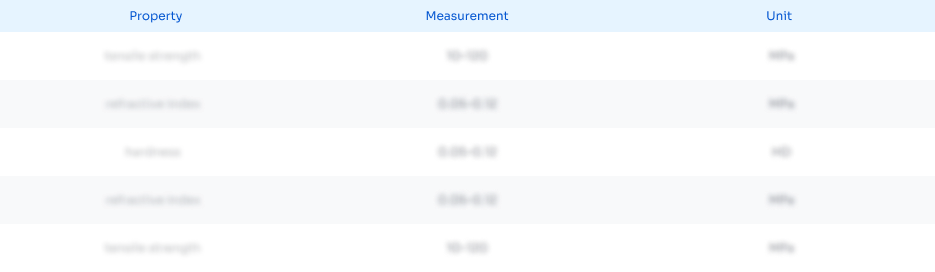
Abstract
Description
Claims
Application Information
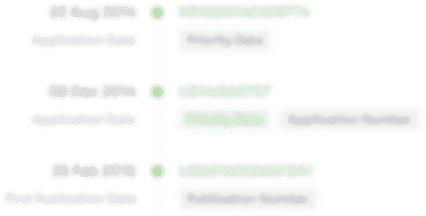
- Generate Ideas
- Intellectual Property
- Life Sciences
- Materials
- Tech Scout
- Unparalleled Data Quality
- Higher Quality Content
- 60% Fewer Hallucinations
Browse by: Latest US Patents, China's latest patents, Technical Efficacy Thesaurus, Application Domain, Technology Topic, Popular Technical Reports.
© 2025 PatSnap. All rights reserved.Legal|Privacy policy|Modern Slavery Act Transparency Statement|Sitemap|About US| Contact US: help@patsnap.com