A Color Image Semantic Classification Method Based on Fully Convolutional Network
A fully convolutional network, color image technology, applied in the field of color semantic classification of color images, to achieve the effect of improving the training speed, improving the accuracy of color semantic classification, and increasing the type and quantity
- Summary
- Abstract
- Description
- Claims
- Application Information
AI Technical Summary
Problems solved by technology
Method used
Image
Examples
Embodiment Construction
[0048] like figure 1 As shown, in this embodiment, a color semantic classification method for color images based on a fully convolutional network is performed according to the following steps:
[0049] Step 1. Construct a fully convolutional network for pixel-level color semantic classification of color images I(x, y, k) of any size; the fully convolutional network consists of a convolutional layer, a pooling layer, and a deconvolutional layer ,
[0050] like figure 2 As shown, in this embodiment, the fully convolutional network includes five stages of convolution pooling operations: the first and second stages each include two convolutional layers and one pooling layer; the third, fourth, and fifth stages each It consists of three convolutional layers and one pooling layer. The fully convolutional network has a total of thirteen convolutional layers, five pooling layers, and three deconvolutional layers. The arbitrary size of the color image means that the size of the co...
PUM
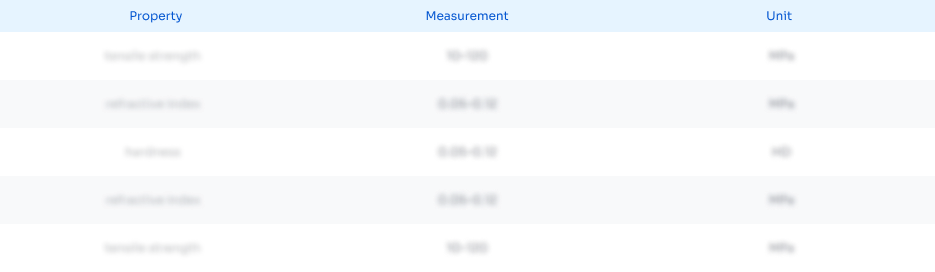
Abstract
Description
Claims
Application Information
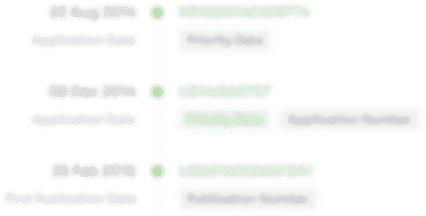
- R&D Engineer
- R&D Manager
- IP Professional
- Industry Leading Data Capabilities
- Powerful AI technology
- Patent DNA Extraction
Browse by: Latest US Patents, China's latest patents, Technical Efficacy Thesaurus, Application Domain, Technology Topic, Popular Technical Reports.
© 2024 PatSnap. All rights reserved.Legal|Privacy policy|Modern Slavery Act Transparency Statement|Sitemap|About US| Contact US: help@patsnap.com