Hierarchical latent variable model-based news recommendation method
A recommendation method and hidden variable technology, applied in special data processing applications, instruments, electrical digital data processing, etc., can solve the problems of sparse user item rating matrix, lack of novelty, inaccurate recommendation, etc., achieve good real-time performance, prevent Overfitting, high scalability effects
- Summary
- Abstract
- Description
- Claims
- Application Information
AI Technical Summary
Problems solved by technology
Method used
Image
Examples
Embodiment
[0046] The idea of threshold autoregression is to divide the entire space into several small spaces, consider a linear model in each small space, and then combine these small models together to form a model on the entire space. In the entire hybrid model, this application divides user characteristics and item characteristics into several subcategories, establishes a hidden variable model on each category, and then combines these several categories together to form the entire hidden variable model, which is called subclassification. Layer hidden variable model.
[0047] Such as figure 1 Shown is the block flow chart of the present invention, and the present invention mainly comprises seven big steps.
[0048] S1. News crawling. According to the structure of different portal websites, configure different regular expressions to crawl different types of news from major portal websites and store them in the local database of the recommendation system. The crawled content include...
PUM
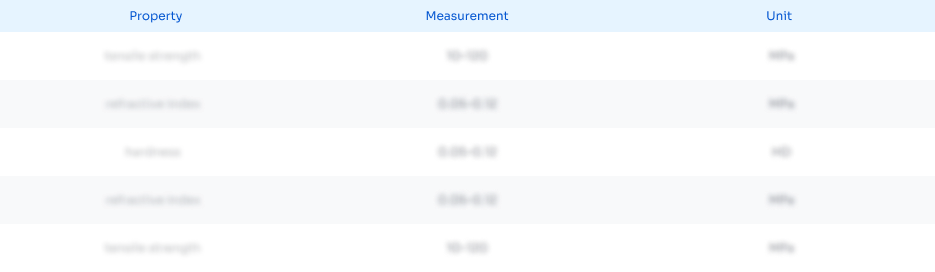
Abstract
Description
Claims
Application Information
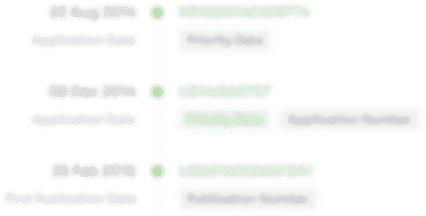
- R&D Engineer
- R&D Manager
- IP Professional
- Industry Leading Data Capabilities
- Powerful AI technology
- Patent DNA Extraction
Browse by: Latest US Patents, China's latest patents, Technical Efficacy Thesaurus, Application Domain, Technology Topic.
© 2024 PatSnap. All rights reserved.Legal|Privacy policy|Modern Slavery Act Transparency Statement|Sitemap