Mass spatial data density clustering method based on elastic distribution dataset
A spatial data and elastic distribution technology, applied in structured data retrieval, electronic digital data processing, geographic information database, etc., can solve the problems of high algorithm I/O overhead, inability to use real-time clustering, high delay, etc. The effect of delayed, fast clustering
- Summary
- Abstract
- Description
- Claims
- Application Information
AI Technical Summary
Problems solved by technology
Method used
Image
Examples
Embodiment Construction
[0036] The present invention is further described below in conjunction with specific embodiment, but protection scope of the present invention is not limited thereto:
[0037] Embodiment: In the present embodiment, suppose D={p 1 ,p 2 ,...,p n} is the data set to be clustered, and its k-dimensional space area S is the calculation space of D. is a data object in k-dimensional space (1≤i≤n), for p i Projection on the k-th dimension axis. A mass spatial data density clustering method based on elastic distribution data sets The main steps of clustering are as follows: figure 1 Shown, the realization of the present invention is based on following basic concepts:
[0038] Definition 1 (dc neighborhood): with a given data object p i As the center, the k-dimensional space within its radius dc is called p i The dc neighborhood. For the data object p in the dc neighborhood j , with dist(p i ,p j )<dc.
[0039] Definition 2 (local density p i ):p i The number of data obje...
PUM
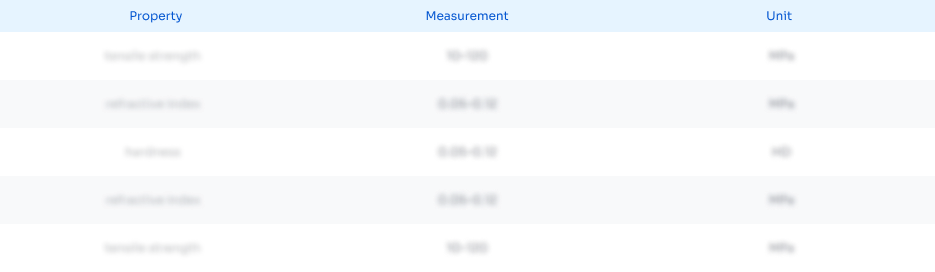
Abstract
Description
Claims
Application Information
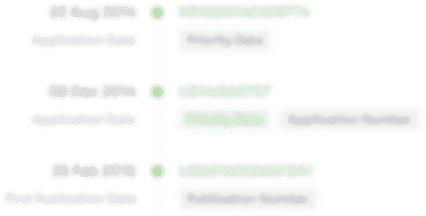
- R&D Engineer
- R&D Manager
- IP Professional
- Industry Leading Data Capabilities
- Powerful AI technology
- Patent DNA Extraction
Browse by: Latest US Patents, China's latest patents, Technical Efficacy Thesaurus, Application Domain, Technology Topic, Popular Technical Reports.
© 2024 PatSnap. All rights reserved.Legal|Privacy policy|Modern Slavery Act Transparency Statement|Sitemap|About US| Contact US: help@patsnap.com