Association rule-based multi-tag Chinese emotion classification method
A sentiment classification and multi-label technology, which is applied in text database clustering/classification, semantic analysis, unstructured text data retrieval, etc., can solve problems such as poor classification effect of multi-label learning algorithms, and achieve the effect of improving mining performance
- Summary
- Abstract
- Description
- Claims
- Application Information
AI Technical Summary
Problems solved by technology
Method used
Image
Examples
Embodiment Construction
[0016] combine figure 1 , a multi-label Chinese sentiment classification method based on association rules, including the following steps:
[0017] Step 1, using the association rule algorithm to find frequent itemsets between various Chinese emotional markers;
[0018] Step 2, derive association rules between emotion tags according to frequent itemsets;
[0019] Step 3, modify the multi-label data set using association rules to obtain new data;
[0020] Step 4, use the Rank-SVM algorithm to classify the obtained new data set and learn to obtain a new model;
[0021] Step 5, use the new model to make predictions on the test dataset.
[0022] In step 1, the specific process of using the association rule algorithm to find frequent itemsets between various Chinese sentiment tags is as follows:
[0023] Step S100, set the Chinese sentiment tag set [y 1 ,y 2 ,...,y n ], the sentiment label set l corresponding to the ith example in the dataset i , generate a row vector v=[v ...
PUM
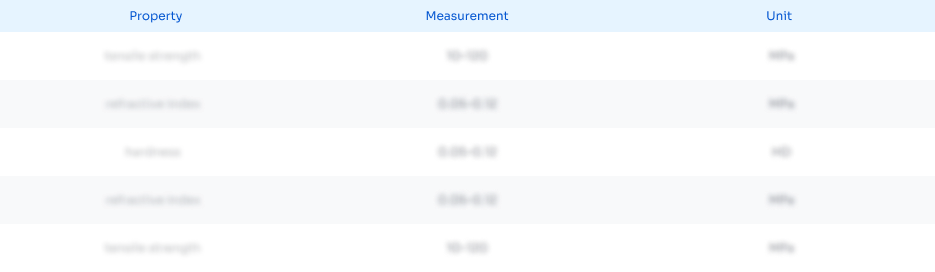
Abstract
Description
Claims
Application Information
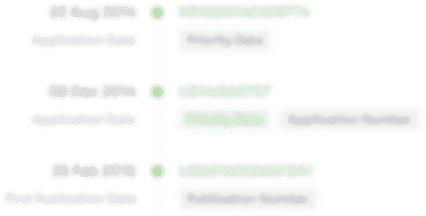
- R&D Engineer
- R&D Manager
- IP Professional
- Industry Leading Data Capabilities
- Powerful AI technology
- Patent DNA Extraction
Browse by: Latest US Patents, China's latest patents, Technical Efficacy Thesaurus, Application Domain, Technology Topic, Popular Technical Reports.
© 2024 PatSnap. All rights reserved.Legal|Privacy policy|Modern Slavery Act Transparency Statement|Sitemap|About US| Contact US: help@patsnap.com