Adaptive simulated annealing genetic algorithm used for sleep electroencephalogram staging feature selection
A genetic algorithm and simulated annealing technology, applied in the direction of genetic rules, calculation, application, etc., can solve the problems of great influence on the stability of the solution, slow convergence speed, non-convergence of iteration results, etc., and achieve excellent feature screening effect and correct classification high rate effect
- Summary
- Abstract
- Description
- Claims
- Application Information
AI Technical Summary
Problems solved by technology
Method used
Image
Examples
Embodiment Construction
[0033] The present invention will be described in further detail below in conjunction with the accompanying drawings: the present embodiment is implemented on the premise of the technical solution of the present invention, and detailed implementation is provided, but the protection scope of the present invention is not limited to the following embodiments.
[0034] An adaptive simulated annealing genetic algorithm for sleep EEG staging feature selection involved in this embodiment, firstly, the adaptive adjustment mechanism of the adaptive genetic algorithm crossover probability and mutation probability is added to the simulated annealing genetic algorithm; secondly, The genetic optimization algorithm is used to replace the neighborhood random selection mechanism in the simulated annealing genetic algorithm; finally, a weighted fitness function is designed to ensure the accuracy and similarity of the classification results.
[0035] Specific steps are as follows:
[0036] Step...
PUM
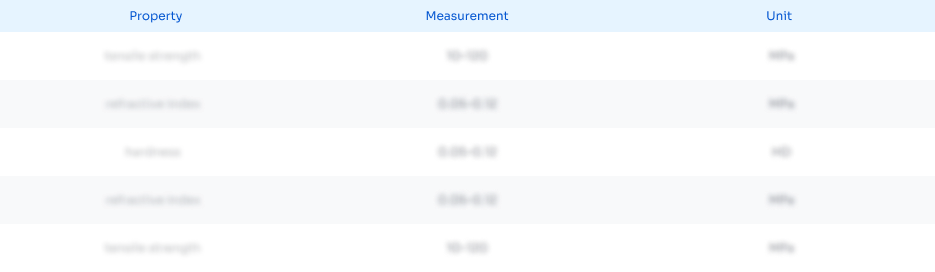
Abstract
Description
Claims
Application Information
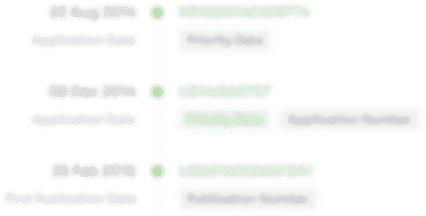
- R&D Engineer
- R&D Manager
- IP Professional
- Industry Leading Data Capabilities
- Powerful AI technology
- Patent DNA Extraction
Browse by: Latest US Patents, China's latest patents, Technical Efficacy Thesaurus, Application Domain, Technology Topic, Popular Technical Reports.
© 2024 PatSnap. All rights reserved.Legal|Privacy policy|Modern Slavery Act Transparency Statement|Sitemap|About US| Contact US: help@patsnap.com