Nonnegative matrix factorization-based method for detecting anomalies in stock market
A non-negative matrix decomposition and anomaly detection technology, applied in the field of anomaly detection, can solve the problem of high average error rate of outliers
- Summary
- Abstract
- Description
- Claims
- Application Information
AI Technical Summary
Problems solved by technology
Method used
Image
Examples
experiment example
[0106] We collected the Shanghai stock index data from 2000 / 01 / 04 to 2015 / 12 / 03, with a total of 3851 records and 42 attribute values. Corresponding to 42 15-year time series stock indexes. Then, perform a non-negative matrix factorization of X m×n =U m×r V r×n , r is the decomposition index. Among them, U represents the base matrix, and each column vector is the primitive to construct the entire stock index matrix; V represents the coefficient matrix, which is the weight of the primitive to construct the stock index. We use the weight sequence to represent the entire stock index sequence; r also represents the feature space the degree of compression. r=n, then there is no compression during decomposition. In the experiment, three sets of decomposed coefficient matrices obtained by r=1, 5, and 10 are used to represent the original sampling data in a compressed manner.
[0107] Since the decomposition adopts an iterative decomposition method, that is, the base matrix U is...
PUM
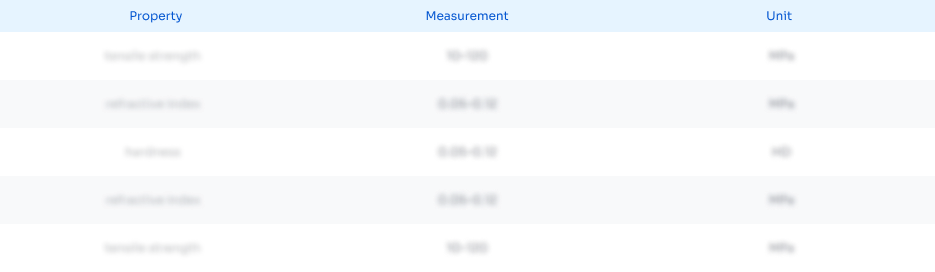
Abstract
Description
Claims
Application Information
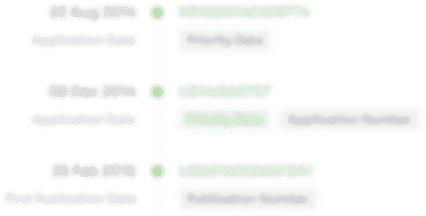
- R&D Engineer
- R&D Manager
- IP Professional
- Industry Leading Data Capabilities
- Powerful AI technology
- Patent DNA Extraction
Browse by: Latest US Patents, China's latest patents, Technical Efficacy Thesaurus, Application Domain, Technology Topic, Popular Technical Reports.
© 2024 PatSnap. All rights reserved.Legal|Privacy policy|Modern Slavery Act Transparency Statement|Sitemap|About US| Contact US: help@patsnap.com