Multi-source remote sensing image surface object classification method based on double-channel convolution step network
A technology for classification of remote sensing images and features, applied in the field of classification of multi-source remote sensing images and features, can solve the problems of consuming manpower and financial resources and high costs, and achieve improved classification accuracy, high classification accuracy and representative effects
- Summary
- Abstract
- Description
- Claims
- Application Information
AI Technical Summary
Problems solved by technology
Method used
Image
Examples
Embodiment Construction
[0042] The present invention will be further described below in conjunction with the accompanying drawings.
[0043] see figure 1 , the concrete implementation steps of the present invention are as follows:
[0044] Step 1. The multispectral data of the five cities of Berlin, Paris, Hong Kong, Rome, and Sao Paulo obtained by the landsat-8 sensor were normalized using ENVI software, and the normalized multispectral data were obtained, which were recorded as landsat_berlin , landsat_paris, landsat_hong_kong, landsat_rome, landsat_sao_paulo;
[0045] The multispectral data of the five cities of Berlin, Paris, Hong Kong, Rome, and Sao Paulo obtained by the landsat-8 sensor are all 9 bands, and the image sizes are 666×643, 988×1160, 529×528, 447×377, 871× 1067;
[0046] When using ENVI software to normalize the data, the selected normalization method is equalize;
[0047] Step 2: Normalize the multispectral data of the five cities of Berlin, Paris, Hong Kong, Rome, and Sao Paul...
PUM
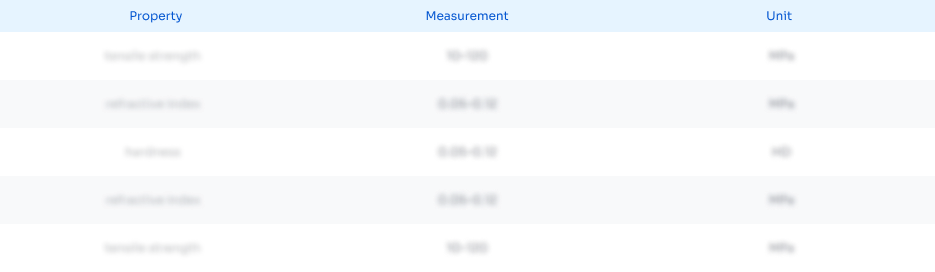
Abstract
Description
Claims
Application Information
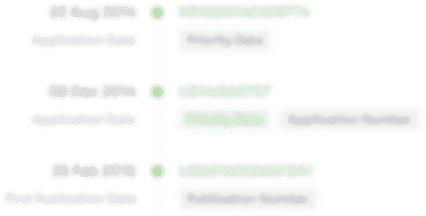
- R&D
- Intellectual Property
- Life Sciences
- Materials
- Tech Scout
- Unparalleled Data Quality
- Higher Quality Content
- 60% Fewer Hallucinations
Browse by: Latest US Patents, China's latest patents, Technical Efficacy Thesaurus, Application Domain, Technology Topic, Popular Technical Reports.
© 2025 PatSnap. All rights reserved.Legal|Privacy policy|Modern Slavery Act Transparency Statement|Sitemap|About US| Contact US: help@patsnap.com