Short-term wind power prediction method based on cloud evolutionary particle swarm algorithm
A technology of wind power prediction and particle swarm algorithm, applied in prediction, calculation, instrument and other directions, can solve problems such as instability, particle fitness value changes greatly, particles are difficult to converge quickly, etc., to improve prediction accuracy and efficient operation. Effect
- Summary
- Abstract
- Description
- Claims
- Application Information
AI Technical Summary
Problems solved by technology
Method used
Image
Examples
Embodiment Construction
[0036] According to the attached figure 1 and figure 2 , give a preferred embodiment of the present invention, and give a detailed description, so that the functions and characteristics of the present invention can be better understood.
[0037] see figure 1 and figure 2 , the present invention provides a short-term wind power prediction method based on cloud evolutionary particle swarm algorithm, comprising steps:
[0038] S1: Establish a feed-forward neural network prediction model 1.
[0039] The feedforward neural network model includes an input layer 11 , a hidden layer 12 and an output layer 13 . The training problem of feedforward neural network prediction model 1 is essentially a complex continuous parameter optimization problem, that is, to find the optimal continuous weight value. In the forward neural network prediction model 1, too large learning rate may cause the algorithm not to converge; too small learning rate will make the algorithm converge very slowl...
PUM
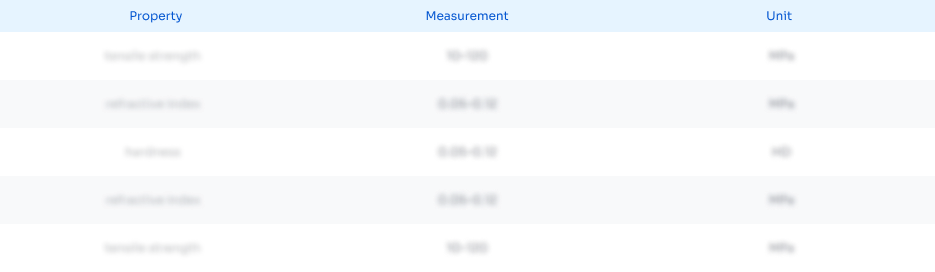
Abstract
Description
Claims
Application Information
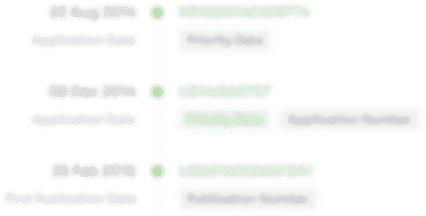
- R&D Engineer
- R&D Manager
- IP Professional
- Industry Leading Data Capabilities
- Powerful AI technology
- Patent DNA Extraction
Browse by: Latest US Patents, China's latest patents, Technical Efficacy Thesaurus, Application Domain, Technology Topic.
© 2024 PatSnap. All rights reserved.Legal|Privacy policy|Modern Slavery Act Transparency Statement|Sitemap