cGAN algorithm-based super-resolution image recovery technology
A super-resolution and image technology, applied in image enhancement, image data processing, graphics and image conversion, etc., can solve problems such as unsatisfactory restoration effect, insufficient model feature weights, poor super-resolution restoration effect of three-channel color images, etc. , to achieve good display effect and short training time
- Summary
- Abstract
- Description
- Claims
- Application Information
AI Technical Summary
Problems solved by technology
Method used
Image
Examples
Embodiment Construction
[0016] The present invention provides a cGAN-based image super-resolution restoration, such as image 3 As shown, it includes the following steps:
[0017] (1) Make training set and image set.
[0018] (2) Design the neural network and adjust it.
[0019] (3) Conduct training.
[0020] (4) Get the model for testing.
[0021] The above 1) create a training set and an image set. Specifically include the following steps:
[0022] 11) Write code through python.
[0023] 12) Download images commonly used in super-resolution research.
[0024] 13) Use the above code to divide the image into uniform sizes.
[0025] 14) Perform low-resolution processing on the image obtained in 13) to obtain two images which are the low-resolution image and the original high-resolution image.
[0026] 2) above design the neural network and tune it. Specifically include the following steps:
[0027] 21) Design neural network deep convolution against neural network DCGAN through Python. The m...
PUM
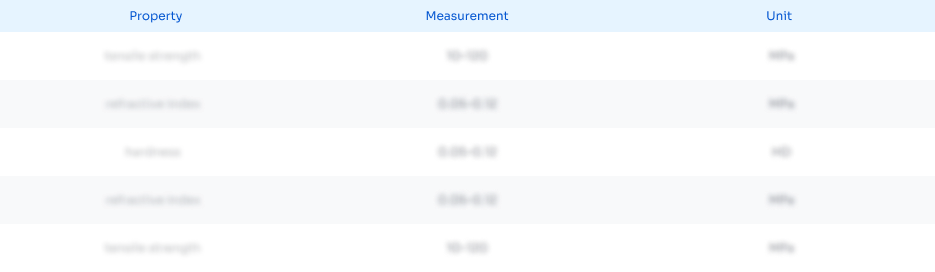
Abstract
Description
Claims
Application Information
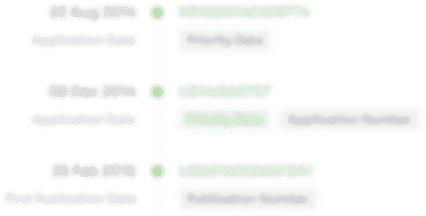
- Generate Ideas
- Intellectual Property
- Life Sciences
- Materials
- Tech Scout
- Unparalleled Data Quality
- Higher Quality Content
- 60% Fewer Hallucinations
Browse by: Latest US Patents, China's latest patents, Technical Efficacy Thesaurus, Application Domain, Technology Topic, Popular Technical Reports.
© 2025 PatSnap. All rights reserved.Legal|Privacy policy|Modern Slavery Act Transparency Statement|Sitemap|About US| Contact US: help@patsnap.com