A Deep Differential Privacy Preservation Method Based on Generative Adversarial Networks
A privacy protection and differential privacy technology, applied in the field of deep learning and privacy protection, it can solve the problem of leaking sensitive user information, and achieve the effect of speeding up training, realizing usability and privacy protection, and reducing the scope of selection.
- Summary
- Abstract
- Description
- Claims
- Application Information
AI Technical Summary
Problems solved by technology
Method used
Image
Examples
Embodiment Construction
[0028] The present invention will be described below in conjunction with the accompanying drawings and specific embodiments. Which attached figure 1 The process of deep differential privacy protection method based on generative adversarial network is described.
[0029] Such as figure 1 Shown, the concrete implementation steps of the present invention:
[0030] (1) Calculate the upper bound of the privacy budget based on the size of the input data set, the query sensitivity, and the probability obtained by the attacker. The calculation method of the upper bound of the privacy budget is:
[0031]
[0032]Where ε is the privacy budget, n is the potential data set of the input data set (the potential data set refers to, assuming the input data set is D, the possible value method of the nearest neighbor data set D' of the data set is n, where D and D 'only one piece of data difference), △q is the sensitivity of the query function q for data sets D and D', △v is the maximum d...
PUM
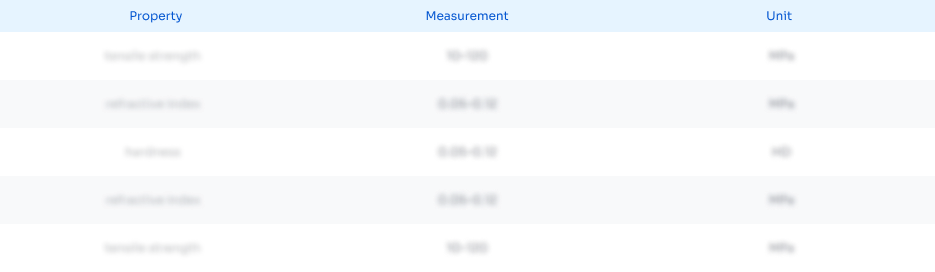
Abstract
Description
Claims
Application Information
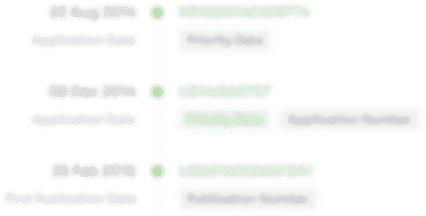
- R&D Engineer
- R&D Manager
- IP Professional
- Industry Leading Data Capabilities
- Powerful AI technology
- Patent DNA Extraction
Browse by: Latest US Patents, China's latest patents, Technical Efficacy Thesaurus, Application Domain, Technology Topic, Popular Technical Reports.
© 2024 PatSnap. All rights reserved.Legal|Privacy policy|Modern Slavery Act Transparency Statement|Sitemap|About US| Contact US: help@patsnap.com