Human face depth and surface normal vector predication method based on dilated convolution neural network
A technology of convolutional neural network and prediction method, applied in the field of computer vision and digital image processing, which can solve the problems of small receptive field of deep network, unable to train, and gradient disappearance.
- Summary
- Abstract
- Description
- Claims
- Application Information
AI Technical Summary
Problems solved by technology
Method used
Image
Examples
Embodiment Construction
[0032] The present invention will be further described below in conjunction with the accompanying drawings and preferred embodiments.
[0033] The specific embodiment of the present invention provides a kind of face depth and surface normal vector prediction method based on hole convolutional neural network, this method comprises the step of training hole convolutional neural network, such as figure 1 Shown, the step of described training hole convolutional neural network comprises S1 to S4:
[0034] S1, build a hole convolution neural network, the hole convolution neural network includes a plurality of convolution layers connected in sequence, a plurality of hole convolution layers and a plurality of deconvolution layers, wherein each of the convolution layers are connected with a normalization operation and an incentive operation;
[0035] S2. Initialize the weight value of the atrous convolutional neural network;
[0036] S3. Input the face pictures in the pre-established...
PUM
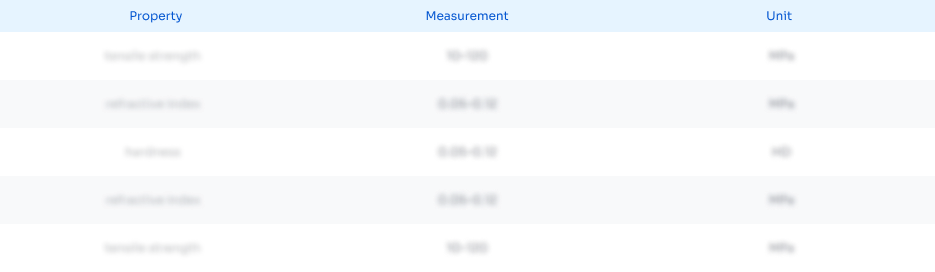
Abstract
Description
Claims
Application Information
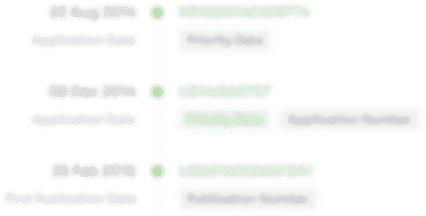
- R&D Engineer
- R&D Manager
- IP Professional
- Industry Leading Data Capabilities
- Powerful AI technology
- Patent DNA Extraction
Browse by: Latest US Patents, China's latest patents, Technical Efficacy Thesaurus, Application Domain, Technology Topic.
© 2024 PatSnap. All rights reserved.Legal|Privacy policy|Modern Slavery Act Transparency Statement|Sitemap