Deep learning based automatic defect identification method for underground pipeline
An underground pipeline, deep learning technology, applied in character and pattern recognition, image data processing, image enhancement and other directions, can solve problems such as insufficient accuracy and slow detection speed, and achieve the effect of improving utilization.
- Summary
- Abstract
- Description
- Claims
- Application Information
AI Technical Summary
Problems solved by technology
Method used
Image
Examples
Embodiment Construction
[0034] The present invention will be further described in detail below in conjunction with the accompanying drawings and examples. The following examples are explanations of the present invention and the present invention is not limited to the following examples.
[0035] Such as figure 1 As shown, a kind of deep learning-based automatic identification method for underground pipeline defects of the present invention is characterized in that it comprises the following steps:
[0036] Step 1: Prepare the underground pipeline positive sample set and negative sample set required for training the convolutional neural network; the positive sample set is a normal and non-defective pipeline image, and the negative sample set is a pipeline image with defects.
[0037] The collected image samples of underground pipelines should be diverse, and the amount of data of defective samples and non-defective samples should be the same. Some training samples such as image 3 shown;
[0038] St...
PUM
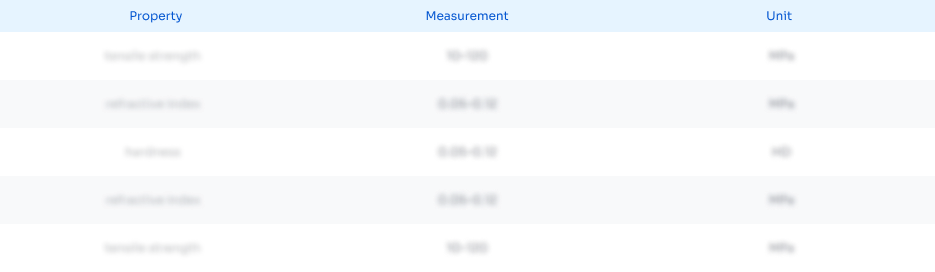
Abstract
Description
Claims
Application Information
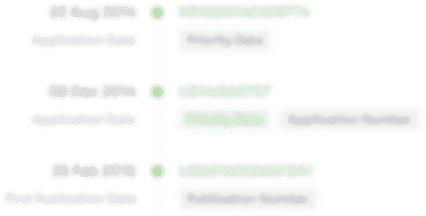
- R&D Engineer
- R&D Manager
- IP Professional
- Industry Leading Data Capabilities
- Powerful AI technology
- Patent DNA Extraction
Browse by: Latest US Patents, China's latest patents, Technical Efficacy Thesaurus, Application Domain, Technology Topic, Popular Technical Reports.
© 2024 PatSnap. All rights reserved.Legal|Privacy policy|Modern Slavery Act Transparency Statement|Sitemap|About US| Contact US: help@patsnap.com