A Transfer Classification Learning Method Preserving the Sparse Structure of Image Classification
A learning method and image technology, applied in the direction of instruments, computing, character and pattern recognition, etc., can solve the problem that the effect of image classification is not very satisfactory, and achieve the effect of solving unsatisfactory results and improving performance
- Summary
- Abstract
- Description
- Claims
- Application Information
AI Technical Summary
Problems solved by technology
Method used
Image
Examples
Embodiment Construction
[0081] Below in conjunction with accompanying drawing, technical scheme of the present invention is described in further detail:
[0082] like figure 1 As shown, the present invention discloses a migration classification learning method that maintains the sparse structure of image classification, comprising the following steps:
[0083] Step 1), collect source domain image data, source domain image labels and target domain image data, the feature space of the source domain image data and target domain image data is the same: if both collect the grayscale of each pixel in each image As each feature value, the distribution of the source domain image data and the target domain image data are required to be different. For example, the shooting lighting conditions of the source domain image and the target domain image are different, and the labels (categories) contained in the source domain and the target domain are required to be consistent. .
[0084] Step 2), using methods suc...
PUM
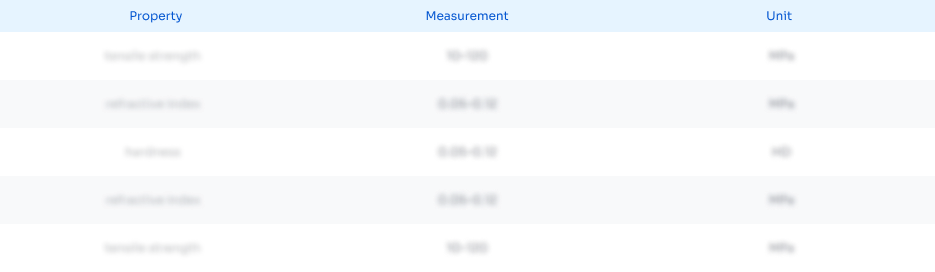
Abstract
Description
Claims
Application Information
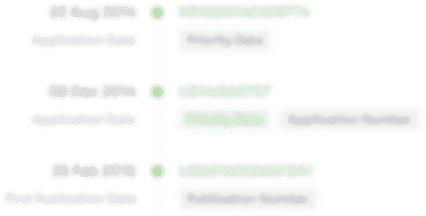
- R&D
- Intellectual Property
- Life Sciences
- Materials
- Tech Scout
- Unparalleled Data Quality
- Higher Quality Content
- 60% Fewer Hallucinations
Browse by: Latest US Patents, China's latest patents, Technical Efficacy Thesaurus, Application Domain, Technology Topic, Popular Technical Reports.
© 2025 PatSnap. All rights reserved.Legal|Privacy policy|Modern Slavery Act Transparency Statement|Sitemap|About US| Contact US: help@patsnap.com