Graph-structured-data generation method based on deep convolutional generative adversarial networks (DCGAN)
A technology of data generation and deep convolution, applied in the field of big data, can solve the problems of deviation, simulation graph structure data cannot have multiple attributes, etc., and achieve the effect of easy feature extraction and obvious characteristics
- Summary
- Abstract
- Description
- Claims
- Application Information
AI Technical Summary
Problems solved by technology
Method used
Image
Examples
Embodiment Construction
[0047] In order to make the object, technical solution and advantages of the present invention clearer, the present invention will be further described in detail below in conjunction with the accompanying drawings and embodiments. It should be understood that the specific embodiments described here are only used to explain the present invention, not to limit the present invention. In addition, the technical features involved in the various embodiments of the present invention described below can be combined with each other as long as they do not constitute a conflict with each other.
[0048] First introduce the deep convolutional generation confrontation network. The deep convolutional generation confrontation network (Deep Convolutional Generative Adversarial Networks, DCGAN) is a generation confrontation network proposed in 2015. The network mainly includes two parts: generator G(.) and discriminator D(.). The generator G(.) deconvolutes the input noise vector Z to generat...
PUM
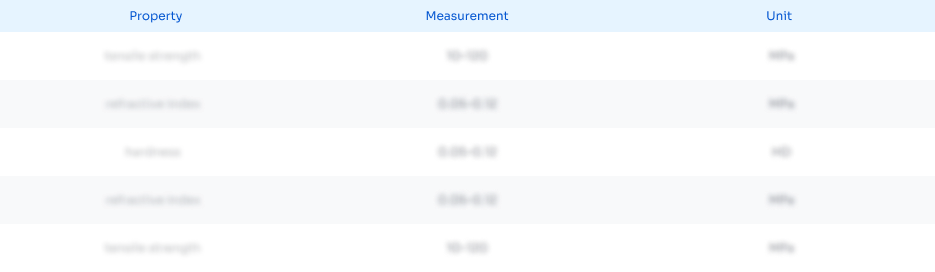
Abstract
Description
Claims
Application Information
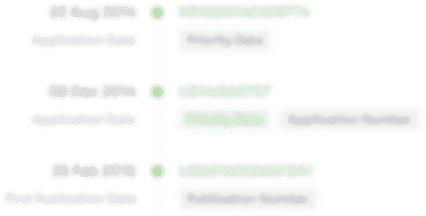
- R&D Engineer
- R&D Manager
- IP Professional
- Industry Leading Data Capabilities
- Powerful AI technology
- Patent DNA Extraction
Browse by: Latest US Patents, China's latest patents, Technical Efficacy Thesaurus, Application Domain, Technology Topic.
© 2024 PatSnap. All rights reserved.Legal|Privacy policy|Modern Slavery Act Transparency Statement|Sitemap