Email binary classification algorithm based on active learning and negative selection
A technology of negative selection and classification algorithm, applied in text database clustering/classification, computing, computer components, etc., can solve the problems of low generalization performance, high economic cost, and large impact on classification results, and achieve accelerated mail classification process, improve classification accuracy, and reduce the effect of CPU processing time
- Summary
- Abstract
- Description
- Claims
- Application Information
AI Technical Summary
Problems solved by technology
Method used
Image
Examples
Embodiment Construction
[0047] In order to make the objectives, technical solutions, and advantages of the present invention clearer, the following further describes the present invention in detail with reference to the accompanying drawings and embodiments. It should be understood that the specific embodiments described here are only used to explain the present invention, but not to limit the present invention.
[0048] The two-class mail classification algorithm based on active learning and negative selection proposed by the present invention includes the following steps:
[0049] S1. Utilize a mail set consisting of legitimate mail and spam S 0 Establish the user’s positive interest set P and negative interest set N, and The specific process is as follows:
[0050] S11, eliminate S 0j (S 0j ∈S 0 , 1≤j≤|S 0 |, |S 0 | Means S 0 The number of elements in the set) in the attachments, tags, punctuation marks, special symbols, and stop words, the remaining text is segmented and the root is restored to form S ...
PUM
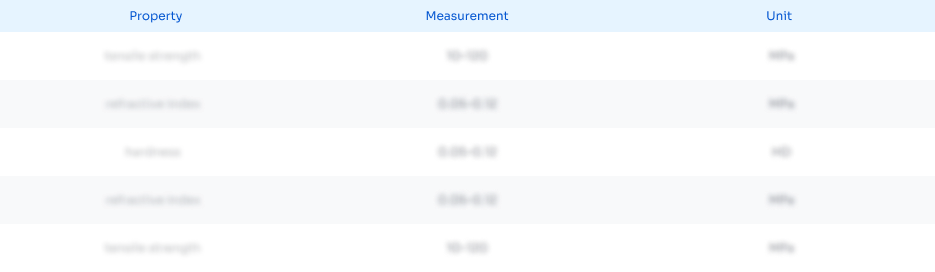
Abstract
Description
Claims
Application Information
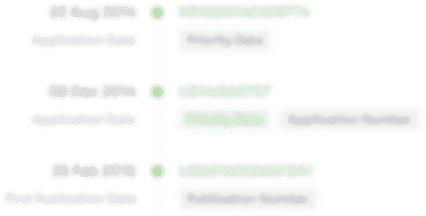
- R&D Engineer
- R&D Manager
- IP Professional
- Industry Leading Data Capabilities
- Powerful AI technology
- Patent DNA Extraction
Browse by: Latest US Patents, China's latest patents, Technical Efficacy Thesaurus, Application Domain, Technology Topic.
© 2024 PatSnap. All rights reserved.Legal|Privacy policy|Modern Slavery Act Transparency Statement|Sitemap