Infant brain magnetic resonance image partitioning method based on fully convolutional network
A magnetic resonance image and convolutional network technology, applied in image analysis, image enhancement, image data processing, etc.
- Summary
- Abstract
- Description
- Claims
- Application Information
AI Technical Summary
Problems solved by technology
Method used
Image
Examples
Embodiment Construction
[0034]It should be noted that, in the case of no conflict, the embodiments in the present application and the features in the embodiments can be combined with each other. The present invention will be further described in detail below in conjunction with the drawings and specific embodiments.
[0035] figure 1 It is a system framework diagram of a method for segmenting infant brain magnetic resonance images based on a fully convolutional network in the present invention. It mainly includes multi-stream 3D fully convolutional network (FCN) with skip connections, partial transfer learning, training, testing and evaluation.
[0036] In partial transfer learning, the weights of shallow layers in deep neural networks are general, while the weights of deep layers are more task-specific; in order to better take advantage of transfer learning, it is necessary to transfer from a training model of a related task; use A pre-trained model designed to segment the proximal femur from 3D T1...
PUM
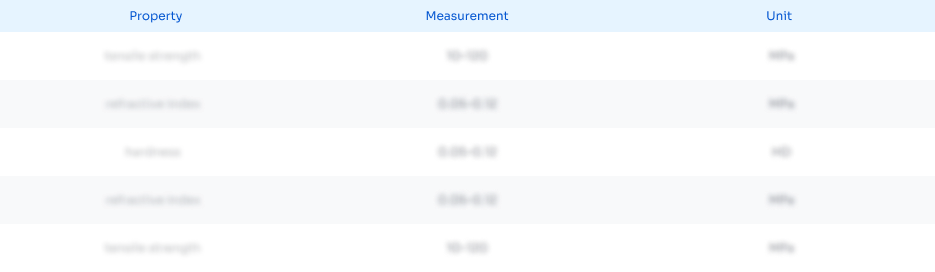
Abstract
Description
Claims
Application Information
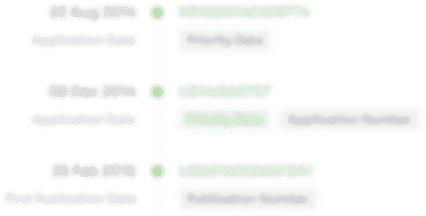
- R&D Engineer
- R&D Manager
- IP Professional
- Industry Leading Data Capabilities
- Powerful AI technology
- Patent DNA Extraction
Browse by: Latest US Patents, China's latest patents, Technical Efficacy Thesaurus, Application Domain, Technology Topic, Popular Technical Reports.
© 2024 PatSnap. All rights reserved.Legal|Privacy policy|Modern Slavery Act Transparency Statement|Sitemap|About US| Contact US: help@patsnap.com