Multi-variable time sequence classifying method based on semantic selection
A technology of time series and classification methods, applied in text database clustering/classification, special data processing applications, instruments, etc., can solve the problem of inability to achieve sample extraction, time dependence is not considered, manual design of features is time-consuming and error-prone, etc. question
- Summary
- Abstract
- Description
- Claims
- Application Information
AI Technical Summary
Problems solved by technology
Method used
Image
Examples
Embodiment
[0038] As a popular research branch of machine learning, time series classification has broad application prospects, such as computer vision, financial analysis, biostatistics, and so on. The Sparse and Adaptive Semantics Learning Network (SA-SLN) proposed in the present invention uses a sparse convolution kernel to simultaneously extract the semantic concepts and temporal short-term dependencies of multivariate time series, and proposes a The attention shift method is used to select semantic concepts, and finally the long-term dependence of the sequence is modeled through LSTMs. The existing time series classification methods need to rely on artificial feature engineering design features or semi-automatically extract spatial features, and use the same feature extraction method for different samples in the same set. In this embodiment, a sparse rule-constrained convolution kernel is used to implement fully automatic semantic concept extraction, and an attention mechanism is com...
Embodiment 2
[0061] figure 1 Is the flow chart of this embodiment, such as figure 1 As shown, this embodiment discloses a multivariate time series classification method based on semantic selection, and the method specifically includes the following steps:
[0062] S1. Collect UTD-MHAD action sequence data sets;
[0063] S2. Because it is a skeleton node action sequence data set, the decentralization operation is performed with the average of the coordinates of the left and right hips and the center node as the origin, and padded with 0 to 121 frames and smoothed filtering to remove noise. Finally, follow the verification method defined in the literature and conduct a cross-topic verification method, and divide the 861 samples of the complete set into a training set of 431 samples and a test set of 430 samples;
[0064] S3, such as figure 2 As shown, the action sequence can be regarded as a high-dimensional multi-variable time sequence. The sequence is organized into a matrix form, and the spar...
PUM
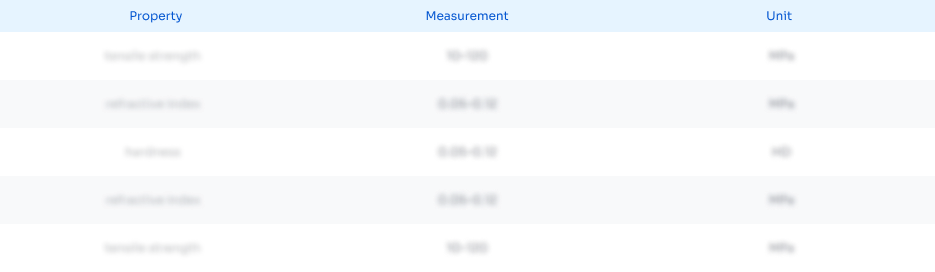
Abstract
Description
Claims
Application Information
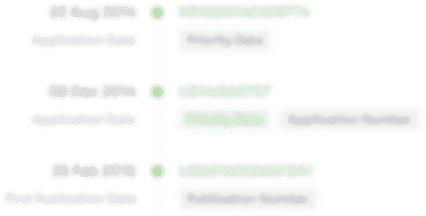
- R&D Engineer
- R&D Manager
- IP Professional
- Industry Leading Data Capabilities
- Powerful AI technology
- Patent DNA Extraction
Browse by: Latest US Patents, China's latest patents, Technical Efficacy Thesaurus, Application Domain, Technology Topic, Popular Technical Reports.
© 2024 PatSnap. All rights reserved.Legal|Privacy policy|Modern Slavery Act Transparency Statement|Sitemap|About US| Contact US: help@patsnap.com